the Creative Commons Attribution 4.0 License.
the Creative Commons Attribution 4.0 License.
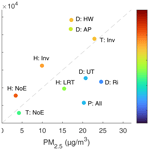
Comparison of size distribution and electrical particle sensor measurement methods for particle lung deposited surface area (LDSAal) in ambient measurements with varying conditions
Henna Lintusaari
Laura Salo
Ville Silvonen
Luis M. F. Barreira
Jussi Hoivala
Lassi Markkula
Jarkko V. Niemi
Jakub Ondracek
Kimmo Teinilä
Hanna E. Manninen
Sanna Saarikoski
Hilkka Timonen
Miikka Dal Maso
Topi Rönkkö
It has become evident that additional metrics along the particle mass concentration, together with dense air quality monitoring networks within cities, are needed to understand the most efficient ways to tackle the health burden of particulate pollution. Particle lung-deposited surface area (LDSAal) is a metric to estimate particle exposure in the lung alveoli, and it has gained interest as a parameter for air quality monitoring as it is relatively easy and cost-efficient to measure with electrical particle sensors. Also, various studies have indicated its potential as a health-relevant metric. In addition to the electrical particle sensors, the LDSAal can be measured with various size distribution methods. However, different LDSAal measurement methods have fundamental differences in their operation principles, e.g., related to the measurement size ranges, size classification or conversion from the originally measured quantity into the LDSAal. It is not well understood how these differences affect the accuracy of the measurement in ambient conditions, where especially the particle effective density and hygroscopicity can considerably change the particle lung deposition efficiencies. In this study, the electrical particle sensor measurement (Partector) and two size distribution approaches (ELPI+ and DMPS/SMPS) were compared in road traffic environments with different environmental conditions in Helsinki and Prague. The results were compared by utilising the general assumptions of the LDSAal measurement (spherical hydrophobic particles with the standard density) and by evaluating the effects of the particle effective density and hygroscopicity. Additionally, the Partector and ELPI+ approaches were compared in various urban environments near road traffic, airports, river traffic and residential wood combustion. The results show that the comparison of different LDSAal measurement methods can be complicated in ambient measurements. The challenges were especially related to the accumulation mode particles roughly larger than 200–400 nm for which the dominant deposition mechanism in the lung changes from diffusion to impaction and the particle effective density and hygroscopicity tend to increase. On the other hand, the results suggest that the differences between the methods are reasonably low when considering only ultrafine and soot particles, which have an effective density closer to the standard (1.0 g cm−3) and are more hydrophobic, highlighting the suitability of the LDSAal as a monitored metric when estimating the spatial differences in the particulate pollution within cities.
- Article
(2955 KB) - Full-text XML
-
Supplement
(1629 KB) - BibTeX
- EndNote
Even though particulate pollution is known to be harmful for human health, it is still not comprehensively understood what the main mechanisms behind the negative health effects are or how to monitor and regulate the health-relevant particulate emissions most efficiently. Since a study by Dockery et al. (1993), the health effects of particulate pollution have been associated especially with fine particulate matter (PM2.5), i.e. the mass concentration of particles smaller than 2.5 µm. PM2.5 is also the most widely used metric for air quality monitoring, regulations and recommendations for ambient particles. However, various studies have indicated that the monitoring of only PM2.5 is not enough in terms of the negative health effects. For example, epidemiological studies have suggested that the dose–response function between PM2.5 and the health effects is not linear, and PM2.5 seems to be relatively more harmful in low-pollution regions compared to highly polluted ones (e.g. Pinault et al., 2016; Vodonos et al., 2018; Strak et al., 2021). Furthermore, in a study by Daellenbach et al. (2020), it was observed that the main sources of particulate matter (PM10; particles smaller than 10 µm) and particle oxidative potential (OP) are not the same in different locations across Europe. Also, PM2.5 toxicity is suggested to be considerably dependent on the emission source and composition (e.g. Jia et al., 2017; Park et al., 2018; Sidwell et al., 2022). All these findings highlight the need for other methods, metrics and points of view, along with the particle mass for regulating and monitoring the particulate pollution to better tackle the adverse health effects of air pollution.
Different particle physical and chemical characteristics likely have a major role in the varying PM2.5 health effects. The chemical composition of particles affects the toxicity and OP of particles as indicated, e.g., by Park et al. (2018) and Daellenbach et al. (2020). Also, the particle size affects the toxicity, which has been suggested to increase as a function of decreasing particle size and increasing surface area concentration (Oberdörster et al., 2005; Schmid and Stoeger, 2016; Hakkarainen et al., 2022). Furthermore, the particle size affects the particle respiratory tract deposition, and especially ultrafine particles, i.e. particles smaller than 100 nm, deposit efficiently in the lung alveoli (ICRP, 1994). The health effects of ultrafine particles are not properly recognised yet, but they have been linked, e.g., to diabetes and myocardial infarction, as well as with changes in the inflammatory status and cardiovascular conditions (Ohlwein et al., 2019; Vallabani et al., 2023). By measuring only PM2.5, the differences in particle composition or in ultrafine particle concentrations cannot properly be detected (e.g. de Jesus et al., 2019; Chen et al., 2022). Thus, equal PM2.5 concentrations in different environments can consist of varying combinations of physical and chemical properties of particles, which likely influences the health effects. Moreover, the composition of particles and ultrafine particle concentrations are typically strongly dependent on the nearby pollution sources, emphasising the need for dense air quality measurement networks (see e.g. Kuula et al., 2020; Edebeli et al., 2023), especially in cities, to better observe and recognise the aerosols that people are exposed to in different locations. For example, it has been suggested that within-city PM2.5 dose–response gradients are steeper than for between-city gradients, emphasising the role of near-source exposure (e.g. to traffic) in terms of adverse health effects of particles (Segersson et al., 2021).
As the current scientific evidence of particle health effects highlights the need for more detailed particle characterisation, as well as for more dense air quality monitoring network within cities, it is crucial to understand what properties of particles should be monitored. Even though the particle chemical composition and OP are likely key factors in the health effects, their utilisation for monitoring purposes is practically challenging due to the expensive and complicated instrumentation (e.g. Onasch et al., 2012). The World Health Organization (WHO) has recommended the measurement of ultrafine particles and black carbon (BC) in the revised air quality guidelines as good practice statements (WHO, 2021). Also, the measurement of the particle lung-deposited surface area (LDSAal) is an interesting option for monitoring measurements. LDSAal measures the surface area concentration of particles that deposit in the lung alveoli, where the interaction between the pulmonary circulation and the respiration occurs. Particles entering the lung alveoli can therefore possibly end up in the blood and other organs like the brain (Heusinkveld et al., 2016). The association between the health effects and LDSAal is not completely known but, e.g., studies by Aguilera et al. (2016) and Patel et al. (2018) indicate that LDSAal has stronger associations with subclinical atherosclerosis and reduced lung function than the particle mass, respectively. Also, LDSAal concentration as a function of PM2.5 has been found to have similar behaviour as the PM2.5 dose–response function, which highlights the potential of the metric in terms of the health effects (Lepistö et al., 2023). It is worth noting that, in different studies, LDSA can also be referred to when considering other respiratory tract regions than the alveoli (e.g. Liu et al., 2023). Here, the notation of LDSAal is used to clarify that only alveolar deposition is considered in this study (see Lepistö et al., 2023).
For air quality monitoring, LDSAal is a convenient metric (e.g. Kuula et al., 2020; Edebeli et al., 2023) as it is reasonably easy to measure with electrical particle sensors such as the Partector (Fierz et al., 2014), nanoparticle surface area monitor (NSAM; Shin et al., 2007), Aerasense MP (Marra et al., 2019) and Pegasor PPS-M (Järvinen et al., 2015). In addition, LDSAal is strongly affected by local emissions of ultrafine particles (Liu et al., 2023; Lepistö et al., 2023) and BC (e.g. Reche et al., 2015; Kuula et al., 2020; Lepistö et al., 2022), which both have been indicated to be important health-relevant parameters (e.g. Janssen et al., 2011; WHO, 2021). Therefore, the electrical particle sensor measurements of LDSAal could provide a relatively easy and cost-efficient method to monitor local particulate pollution with a dense monitoring network within cities.
In addition to the electrical particle sensors, LDSAal can be measured with a size-distribution-based measurement, where the obtained size distributions are weighted with the particle lung deposition efficiency function. For example, the scanning/differential mobility particle sizer (SMPS/DMPS) and the electrical low-pressure impactor (ELPI+) have been utilised in the LDSAal measurements (e.g. Lepistö et al., 2020; Teinilä et al., 2022; Liu et al., 2023; Chen et al., 2023; Lepistö et al., 2023). These three approaches have major differences in their fundamental operation principles. For example, the electrical methods (sensors and the ELPI+) determine the surface area based on the electric charge of particles after a diffusion charger (proportional to particle size), whereas, with the SMPS/DMPS, the surface area is determined based on the particle number size distribution, e.g. by assuming spherical particles. Also, the electrical particle sensors measure LDSAal by assuming certain particle size in the calibration, and they measure LDSAal with a reasonable accuracy only up to roughly 400 nm (Todea et al., 2015). Furthermore, with the size distribution methods, particle size classification depends on different particle concepts with the ELPI+ (aerodynamic diameter) and the SMPS/DMPS (mobility equivalent diameter), and, hence, assumptions of the particle effective density cause uncertainty between the methods if additional instrumentation is not available to determine the effective density accurately. Even though these limitations of the instruments are generally well known, the differences in the reported LDSAal concentrations or size distributions with the different methods in varying ambient conditions are poorly understood.
Also, a better understanding of how well the different methods actually measure the particle lung deposition is needed. As mentioned, the size distribution methods need assumptions of particle effective density, which causes uncertainty in the devices' operation. However, the effective density also affects the particle lung deposition efficiency which increases the vulnerability to errors even more in LDSAal measurement. Moreover, the hygroscopic growth of particles in the lungs is often neglected in LDSAal measurements as it requires detailed information of the particle composition. Both the effective density and the hygroscopic growth can considerably change the particle lung deposition efficiencies (e.g. Löndahl et al., 2014; Vu et al., 2015; Lizonova et al., 2024). These factors are, however, practically challenging to consider in air quality monitoring measurements as they require additional instrumentation which cannot be considered a realistic approach with dense air quality monitoring networks. Also, with the electrical particle sensor measurement, these factors cannot be taken into account. In addition, particle lung deposition efficiencies are individual and dependent on the human anatomy and the breathing pattern, and, thus, approximations of the exposed population are always needed.
To understand the suitability of the LDSAal measurement in air quality monitoring measurements, first, it is important to know how the different measurement methods compare with each other. Second, it is crucial to know how well the different methods, with their typical measurement assumptions, actually measure the particle lung deposition and whether there are variations in the performances of devices in different locations and conditions.
In this study, LDSAal measurements with an electrical particle sensor (Partector), an ELPI+ and a mobility particle sizer (DMPS or SMPS) were compared at road traffic sites with varying conditions in Helsinki (Finland) and Prague (Czechia). The aim was to understand how well the different measurement methods compare with each other and how vulnerable they are to errors in the estimated lung deposition due to the assumptions related to the particle effective density and hygroscopicity. Furthermore, reported LDSAal concentrations with the Partector and ELPI+ were compared to additional measurements in Tampere (Finland) and Düsseldorf (Germany), including road and river traffic, airports and residential wood combustion, to see how they influenced aerosol and to better understand the location-dependent performance of the electrical particle sensor measurement. Ultimately, the study aimed to evaluate the suitability of LDSAal as a metric for air quality monitoring measurements in general and to help the interpretation of previous and future works on ambient LDSAal measurements using different methodologies.
The comparisons with the three different LDSAal measurement methods (Partector, ELPI+ and DMPS/SMPS) were conducted in road traffic environments in Helsinki (Finland) and Prague (Czechia). The DMPS was used to measure LDSAal in Helsinki, and the SMPS was used in Prague. Furthermore, the Partector and ELPI+ were also compared in different urban environments in Tampere (Finland) and Düsseldorf (Germany), including road and river traffic sites, an airport and a site affected by residential wood combustion near a detached-housing area. Maps of the measurement locations are shown in the Supplement (Figs. S1–S4).
2.1 Instrumentation
The Partector (Naneos Particle Solutions GmbH; Fierz et al., 2014) represents the electrical particle sensor measurement method for LDSAal, which is based on detecting the electric current caused by the sampled particles after a diffusion charger. The diffusion charging efficiency is determined as a multiplication of the number of elementary charges of a particle after charging (n) and the probability of a particle to penetrate through the charger (P). The product, Pn, is dependent on the particle mobility equivalent size with an exponent varying typically between 1.1 and 1.9 (Dhaniyala et al., 2011; Järvinen et al., 2014). Due to a lucky coincidence, the charger efficiency correlates reasonably well with LDSAal of a single particle in a size range roughly from 20 to 400 nm, which can, however, be altered slightly by adjusting the ion trap voltage of the charger (Fissan et al., 2006). The Partector first charges the sampled particles in a diffusion charger and then converts the detected electric current caused by the sampled particles into the LDSAal concentration with a single calibration factor. The chosen calibration factor is the response coefficient between the electric current and LDSAal at 100 nm, which typically is close to the peak size of the LDSAal size distributions in urban environments (Fierz et al., 2014). A similar approach of converting a diffusion-charged current into LDSAal is also utilised with other sensor-type instruments such as the nanoparticle surface area monitor (NSAM; Shin et al., 2007), Aerasense MP (Marra et al., 2019) and Pegasor PPS-M (Järvinen et al., 2015). The main advantage of the method is that it enables measurement with low-maintenance handheld devices. Also, LDSAal can be determined with 1 s time resolution. On the other hand, the method is reasonably accurate only for particles roughly from 20 to 400 nm, and thus, the accurate performance of the device requires sampled particles to be in a certain size range.
The ELPI+ (Dekati Ltd.; Keskinen et al., 1992; Järvinen et al., 2014) is a particle size distribution measurement device which utilises a 14-stage cascade impactor to classify the sampled particles according to their aerodynamic size. Before the size classification, the sampled particles are charged in a diffusion charger, similar to the electrical sensors. The electric current caused by the collected particles in each impactor stage is measured with electrometers and can then be converted, e.g., to particle number, mass or LDSAal (Lepistö et al., 2020). Each impactor stage uses its own conversion factors for the wanted quantity, depending on the particle size. The 14 stages enable measurement from 6 nm up to 10 µm, and the time resolution of the measurement is 1 s. As the particle charge after the diffusion charger, and, therefore, the measured electric current, is dependent on the particle mobility equivalent diameter, and the size classification is dependent on the aerodynamic diameter, the ELPI+ measurement requires an estimation of the particle effective density to estimate the average electric current caused by a single particle collected onto an impactor stage and to convert the measured current to other quantities accurately.
The DMPS and SMPS both share the same operation principle of measuring the particle size distributions by utilising a combination of a differential mobility analyser (DMA) and a condensation particle counter (CPC). First, the DMA is used to select only certain-sized particles to remain in the sample flow according to their electrical mobility. Then, the remaining sample is measured with the CPC; hence, the number concentration of particles in a certain size range can be determined. By adjusting the DMA parameters, the size range of the measured particles can be changed, enabling the measurement of particle number size distribution. Then, the obtained number size distribution can be weighted with the particle lung deposition function, and thus, the LDSAal concentration and size distribution can be measured. Here, the utilised lung deposition function (similar to the ELPI+ calibration) was based on the ICRP (International Commission on Radiological Protection) model with averaged data for males and females at three physical activity levels: sitting, light exercise and heavy exercise (ICRP, 1994; Hinds, 1999). The ICRP model is a semi-empirical regional compartment lung deposition model which considers the human respiratory tract as a series of filters and utilises measured data with human volunteers. The parameters used in the ICRP model are provided in Table S1 in the Supplement. The size range and resolution, as well as the time resolution of the measurement, depends on the chosen DMA parameters. In general, the time resolution of the method is lower than with the electrical methods. In this study, the DMPS and SMPS measured particles from 10 to 800 nm and from 10 to 500 nm with time resolutions of 520 and 300 s, respectively. The DMPS system (Helsinki) consisted of a Vienna-type DMA and A20 CPC (Airmodus Ltd). The SMPS system (Prague) consisted of EC 3080, DMA 3081 and CPC 3772 (all TSI Incorporated).
In addition to LDSAal measurement devices, an AE33 Aethalometer (Aerosol Magee Scientific; Drinovec et al., 2015) was used to measure the black carbon (BC) concentration, and Teledyne API model T201 was used to measure nitric oxide (NO) concentration during the measurements in Helsinki and Prague.
Differences and challenges with the methods in LDSAal measurement
As none of the described methods directly measures the particle lung deposition, LDSAal is determined with conversion factors from the measured quantity. Generally, the conversion factors into LDSAal are determined by assuming that the measured particles to be spherical with the standard density (1.0 g cm−3) and that the particles do not grow in the human lungs due to hygroscopicity. With the Partector and ELPI+, the measured electric current is converted into LDSAal, whereas, with the DMPS and SMPS, LDSAal is converted from the measured number size distribution.
With the Partector, despite the reasonably good correlation between the electric current and LDSAal with 20–400 nm particles, the needed conversion factor is dependent on the assumed particle size distribution in the calibration; hence, the accuracy is ±30 % in this size range (Todea et al., 2015). LDSAal of particles smaller than 20 nm can generally be assumed to be low due to the small particle size, but the LDSAal of particles larger than 400 nm can greatly be underestimated with the method. For example, in highly polluted environments, the regional aerosol and the accumulation mode particles dominate the particle size distribution, and thus, particles larger than 400 nm can have a significant effect on LDSAal (Salo et al., 2021; Lepistö et al., 2023). Therefore, the performance of the Partector may vary considerably, depending on the dominant pollution source and regional aerosol concentrations.
With the size distribution methods, the size-dependence of the conversion factors can be taken into account, and in principle, varying particle size distributions should not affect the measurement accuracy. However, there are other fundamental challenges with the methods as the ELPI+ measures the size distributions according to the aerodynamic size, whereas the DMPS/SMPS measure them based on the mobility equivalent size. The particle lung deposition is driven by the diffusion with smaller particles (roughly < 0.1 µm) which is dependent on the mobility equivalent size, whereas larger particles (> 0.5 µm) deposit due to impaction and sedimentation, which are depended on the aerodynamic size (Hofmann, 2011). Therefore, the particle effective density not only affects the comparability of the devices, but it also affects the particle lung deposition efficiencies (Löndahl et al., 2014; Lizonova et al., 2024) and, hence, the accuracy of the size distribution methods in terms of LDSAal. Also, it is worth noting that the size ranges of the size distribution measurements vary depending on the study and the device (also in this study), and the studied size range is not typically considered when reporting the LDSAal concentration, even though it can considerably affect the results.
The role of the effective density is also important when considering the conversion from electric current or particle number into LDSAal. As mentioned, in calculation, it is generally assumed that the particles are spherical, but, in reality, ambient particles can have agglomerated and non-spherical structures. On the other hand, the error due to the spherical particle assumption is likely less significant with the electric current measurement as the diffusion-charged current is proportional to particle size and shape, whereas the particle number is not. Therefore, measurement of the electric current may better consider the non-spherical structure of particles in terms of the surface area, which, on the other hand, increases the uncertainty when comparing the different methods (see also Chang et al., 2022; Chen et al., 2023).
Despite the varying operation principles, the different LDSAal measurement methods have shown good agreement with each other in laboratory measurements (e.g. Leavey et al., 2013; Todea et al., 2017; Lepistö et al., 2020), showing that, in principle, all the methods are suitable for measuring LDSAal. However, the comparability of the methods in varying ambient conditions with varying particle characteristics is not well known. For example, Kuula et al. (2019) reported good agreement between the DMPS and various electrical particle sensors at a road traffic site in Helsinki, whereas Chen et al. (2023) observed roughly 1.5 times higher LDSAal concentrations with a NSAM than with a SMPS at a road traffic site in Taiwan. In addition, it should be noted that it is not well known how well the results represent the actual particle lung deposition as, for example, the particle hygroscopicity can considerably change the particle lung deposition efficiency, along with the effective density (Vu et al., 2015). On the other hand, the neglected hygroscopic growth of particles, together with the standard density assumption, is often the only reasonable option for monitoring measurements as the consideration of these parameters requires additional sophisticated instrumentation. Also, in principle, particle lung deposition efficiencies are individual and are dependent on human anatomy and the breathing pattern. Thus, the utilised lung deposition efficiency functions in device calibrations are always approximations, and the chosen approaches may vary with different instruments. Thus, in addition to the uncertainties between the different operation principles of the methods, LDSAal measurements also have uncertainty in the estimation of the actual particle lung deposition.
2.2 Measurement campaigns
2.2.1 Helsinki
In Helsinki, the measurements were conducted during daytime in Mäkelänkatu street canyon in the city centre (60.1963° N, 24.9523° E) on 18 January–16 February 2022. The measurements were done on a kerbside, both in an air quality monitoring supersite operated by Helsinki Region Environmental Services Authority (HSY) and right next to the site in the Aerosol and Trace Gas Mobile Laboratory (ATMo-Lab). The ATMo-Lab is a van which takes the sample above the windshield at the height of 2.2 m and then divides the sample for the instruments located at the back of the vehicle (see, e.g., Lepistö et al., 2023). The street canyon includes three driving lanes in both directions and two tram lines, as well as traffic light junctions. In general, street canyons weaken the dispersion of the road traffic emissions (see the exact characterisation of the same street canyon by Barreira et al., 2021). The ELPI+, Partector, AE33 and T201 measured in the ATMo-Lab, and the DMPS measured in the supersite. The measurements were carried out daily between 06:30 and 19:30 UTC+2 (Helsinki), but the ATMo-Lab was also utilised in driving measurements during the measurement hours which are not considered in the analysis of this study. A detailed description of the measurements is provided by Teinilä et al. (2024).
The conditions during the measurements were typical wintertime conditions in Helsinki, with the average (min–max) temperature, relative humidity and wind speed being −1.5 (−11.1 to 2.9) °C, 88 % (58 %–100 %) and 4.8 (0.6–11.4) m s−1, respectively (Teinilä et al., 2024). On 31 January–5 February an episode of cold weather occurred (temperature below −5 °C) which reduced the dilution and dispersion of pollutants, highlighting the contribution of local emissions within the city. This period is referred to here as an inversion episode. Also, on 13 February a long-range transported (LRT) pollution episode started which lasted until the end of the measurements. During the LRT episode, air masses in Helsinki had travelled through Central and Eastern Europe (Teinilä et al., 2024). This period is referred as a LRT episode in this study.
2.2.2 Prague
The measurements in Prague were carried out during 5 d and 1 night on 25 March–3 April 2022 next to a two-lane street with two tramlines near a train station in Vršovice (50.0664° N, 14.4462° E). In comparison with the Helsinki street canyon site, the measurement site was in an open environment in a preschool yard behind a fence, which limited the direct effects from the nearby traffic. As in Helsinki, the measurements were conducted in a monitoring station and in the ATMo-Lab next to the station. The same ELPI+, Partector, AE33 and T201 units as the ones in Helsinki were installed in the ATMo-Lab, whereas the SMPS measured in the monitoring station. During the studied period, the ATMo-Lab was also utilised in driving measurements and in another measurement location which are not considered in this study. The average (min–max) temperature, relative humidity and wind speed were 6.4 (−1.5–19.4) °C, 65 % (24 %–96 %) and 2.8 (0.3–6.1) m s−1, respectively (data from Helsinki and Prague are provided by the Czech Hydrometeorological Institute).
2.2.3 Additional measurements in Tampere and Düsseldorf
Measurements with the ATMo-Lab, equipped with the same ELPI+ and Partector units, were conducted also in Tampere and Düsseldorf. In Tampere, the measurements were done in an industrial area, which is located next to a train yard, a highway and detached housing areas, on 30 November–20 December 2021. In general, the main source of particles in the measurement site was the road traffic, but during an inversion episode (7–9 December), emissions from the nearby detached housing areas dominated the sampled aerosol. A detailed description of the Tampere measurements is provided by Silvonen et al. (2023). In Düsseldorf, the measurements were carried out in an urban traffic site, on a highway, near an airport and on a riverside of the Rhine during 8–23 March 2022. Detailed descriptions of the Düsseldorf measurements, as well as the ELPI+ LDSAal results, are provided by Lepistö et al. (2023). The data from Düsseldorf were utilised with similar criteria to that found in the corresponding publication.
2.3 Data processing and analysis
Only the data which were measured when all the LDSAal instruments operated in certain environment were considered in the analysis. The presented results are based on the geometric mean of the observed concentrations. With the ELPI+, Partector, AE33 and T201, the data from Helsinki and Prague were, however, first changed to 1 min resolution with an arithmetic mean to reduce noise. With the ELPI+, the upper limit of the measurement size range was 2.5 µm. In addition to LDSAal, the ELPI+ was also used to measure particle number (PN) and PM2.5 concentrations by integrating the obtained particle number and mass size distributions. In Figs. S23 and S25, the LDSAal concentration of particles smaller than 400 nm with the ELPI+ was determined by considering the data from impactor stages 1–7 which correspond to 50 % cut-off diameters starting from 6 to 383 nm. The data from Helsinki and Prague are divided into four categories: (1) measured data in Helsinki that ignore the episodes (Helsinki: No episode), (2) measured data during the inversion episode in Helsinki (Helsinki: Inversion), (3) measured data during the LRT episode in Helsinki (Helsinki: LRT) and (4) all measured data in Prague (Prague: All). The data from Tampere were divided based on the conditions (all data without the inversion episode and during the inversion): (1) No episode and (2) Inversion. The data from Düsseldorf were divided based on the measurement location: (3) Urban traffic, (4) Highway, (5) Airport and (6) River.
With the ELPI+, SMPS and DMPS, LDSAal concentrations and size distributions were determined with three different methods. First, by utilising the general assumptions; i.e. particles have standard effective densities (ρeff = 1 g cm−3), and they do not grow in the human lungs due to hygroscopicity. Second, a sensitivity analysis of the LDSAal calculation was done by correcting the results with an estimated effective density value but not with the hygroscopic growth. Third, the calculation was corrected with estimations of both the particle effective density and the hygroscopic growth. With the Partector, these corrections cannot be applied in the results.
The particle effective density for the sensitivity analysis was estimated by comparing the peak sizes of the surface area size distributions of the ELPI+ to those of the DMPS or SMPS. The relationship between the aerodynamic (da) and the mobility equivalent diameter (dm) is
where Cc is the Cunningham slip correction factor. Hence, the average effective density of particles can be estimated by matching the peak sizes of the surface area size distributions (Figs. S5–S6). The effective density correction (Lepistö et al., 2020) was done by utilising one estimation for the effective density for each studied location, based on the average size distributions of all the measured data in a certain location. The correction calculates the density-corrected deposition function by considering inertial deposition (aerodynamic diameter) and diffusional deposition (mobility equivalent diameter) separately (see ICRP, 1994; Lepistö et al., 2020). The surface area size distribution was chosen for the comparison as it was considered to be the most relevant unit in terms of LDSAal. With this approach, the average effective density in terms of particles contributing to LDSAal can be approximated for a sensitivity analysis, but it should be noted that, in reality, the effective density depends on the particle size and that it has temporal variation. On the other hand, in monitoring measurements, it is not generally possible to monitor the effective density or its size-dependence with a high time resolution, and a representative value which applies for all the observed data must be chosen to support the chosen approach. Also, with the ELPI+, data analysis with a size-dependent effective density is not straightforward due to the cascade impactor measurement. Furthermore, in this study, it was not possible to determine the temporal variation in the effective densities reliably due to the relatively slow SMPS or DMPS measurements.
The effect of particle hygroscopic growth on the particle lung deposition functions was estimated according to the study by Vu et al. (2015) for road traffic environments. The method utilises the data of size-dependent hygroscopic growth ratios of particles observed in road traffic environments which are then taken into account in the lung deposition efficiency calculations by adjusting the particle size. The growth rate is calculated by assuming a relative humidity of 99.5 % in the human lung. It should be noted that the hygroscopicity correction only changes the estimated lung deposition efficiency of particles and not the initial size distribution or the surface area of the inhaled particles. This comparison should be considered an indicative representation of the effects of particle hygroscopic growth in terms of LDSAal measurements, as the particle hygroscopicity is dependent on the particle composition which was not analysed in this study. The approach, however, provides valuable information of the accuracy of the studied methods in terms of the actual particle lung deposition as the particle hygroscopicity has generally been neglected in previous LDSAal studies. The utilised hygroscopicity-corrected lung deposition function (Vu et al., 2015) and the non-corrected one for spherical particles with standard density with the ELPI+ and the DMPS/SMPS data are shown in Fig. S7.
3.1 General overview of the measurements
The average measured PN, PM2.5, NO, and BC concentrations in Helsinki and Prague during the studied periods are shown in Table 1. Also, the estimated average particle effective densities for the sensitivity analysis are shown in the table. In general, the contribution of the nearby road traffic was clearer in Helsinki than in Prague due to the shorter distance from the passing vehicles to the measurement site; this partly explains the relatively higher average PN, NO and BC concentrations compared to PM2.5. In Helsinki, the PM2.5 concentration was mainly low (average of 3.4 µg m−3 without the episodes), indicating low regional pollution in general. In Prague, the average PM2.5 was considerably higher (20.2 µg m−3), which was mainly related to accumulation mode particles and regional aerosol, even though the higher NO also suggests effects from the traffic within the city. In Helsinki, the PM2.5 concentration increased during the inversion and LRT episodes. During the inversion episode, PN, NO and BC concentrations also increased considerably, which indicates local contribution. In addition to road traffic, higher BC during the inversion episode indicates the effects of residential wood combustion, which is typical emission source in Finland during winter (e.g. Teinilä et al., 2022). During the LRT episode, increases with PN, NO and BC were less significant than during the inversion episode when also considering the higher PM2.5, supporting the idea of long-range transported pollution. Histograms of the measured concentrations are provided in Figs. S8–S11.
Table 1Average measured PN, PM2.5, NO and BC concentrations in Helsinki and Prague. Also, the estimation of the average particle effective density (ρeff) for the sensitivity analysis is shown. * The estimated particle effective density in Prague could have been higher based on only the size distribution data. However, a higher ρeff than 2.0 g cm−3 was not considered to be realistic, based on previous studies.
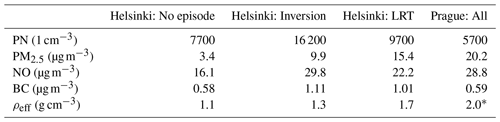
The estimated particle effective densities got higher as the contribution of regional aerosol and PM2.5 concentration increased. In Helsinki, ρeff from local sources (without the episodes) was estimated to be close to the standard. This estimation is supported, e.g., by Virtanen et al. (2006), Rissler et al. (2014) and Wu et al. (2023), who reported effective densities of ultrafine particles of 1.0 (nucleation mode particles), 0.66–1.50 g cm−3 (for 75–100 nm particles) and 0.80–0.89 in road traffic sites in Helsinki, Copenhagen and Taipei, respectively. Also, the increased ρeff due to regional aerosol (i.e. increased PM2.5) is supported by various studies which have reported effective densities of roughly 1.3–2.0 g cm−3, with the average being around 1.5–1.7 g cm−3, for ambient particles in the accumulation mode size ranges (e.g. Virtanen et al., 2006; Levy et al., 2013; Yin et al., 2015; Lu et al., 2024). Interestingly, in Prague, according to the ELPI+ and SMPS data, the estimated ρeff could have been even higher than the chosen 2.0 g cm−3 (Figs. S5–S6). However, the reported effective densities have rarely been higher than 2.0 g cm−3 in previous studies; slightly higher effective densities have been measured that are mainly related to dust episodes or railway emissions (Cha and Olofsson, 2018; Lu et al., 2024). On the other hand, measurements during springtime near a tram line and train station suggest that both dust and railway emissions could have contributed to the measured aerosol in Prague. Still, the main source of the particle surface area was the regional aerosol. Therefore, 2.0 g cm−3 was considered the most realistic estimation for the average effective density in Prague. With this estimation, the surface area size distributions of the ELPI+ and SMPS did not match perfectly (Fig. S5), but this difference is considered to be related to the varying operating principles of the devices and measurement uncertainties. For instance, the measurement of electric current (ELPI+) versus particle number (SMPS) can lead to differences, especially with fractal-structured larger particles, as discussed in Sect. 2.1.1 and later in Sect. 3.2.2. Also, the SMPS upper size limit of 500 nm may have decreased the detection efficiency of 400–500 nm particles.
3.2 LDSAal measurement method comparison
3.2.1 With general assumptions
The measured average LDSAal size distributions and concentrations with general assumptions, i.e. without corrections for the particle effective density and hygroscopicity, are shown in Fig. 1. As can be seen, the mean particle sizes of the LDSAal size distributions were different, which can be explained with the varying aerodynamic and mobility equivalent diameters. Still, in Helsinki, the shapes of the distributions were rather similar with each other, whereas, in Prague, the ELPI+ and SMPS size distributions varied significantly, especially with the accumulation mode particles. In Helsinki, LDSAal concentrations measured with the DMPS and Partector were 69 %–74 % and 76 %–91 % of the ones measured with the ELPI+, respectively. In Prague, LDSAal concentrations with the SMPS and Partector were 54 % and 67 % of the ones measured with the ELPI+. Scatter plots of hourly averaged data are provided in the Supplement (Figs. S16–S18).
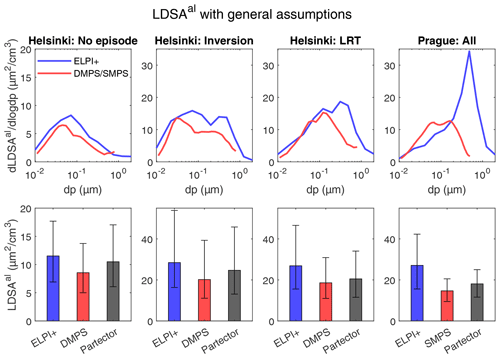
Figure 1Average LDSAal size distributions and concentrations measured with the different methods during the studied periods in Helsinki and Prague without corrections for the particle effective density or the hygroscopic growth. The whiskers indicate the 25th and 75th percentiles of the measured concentrations. LDSAal data are shown in Table S2 (histograms in Figs. S12–S15). Note the different y-axis range for Helsinki: No episode.
Overall, the results in Fig. 1 show that there can be significant differences in the measured LDSAal concentrations with different methods, even in similar kinds of urban environments if the general assumptions are applied with the data. The measured size distributions suggest that this uncertainty is especially related to the estimation of the particle effective density, as the differences with the size distribution methods increased as the ρeff differed from the standard of 1.0 g cm−3. The result in Prague shows that ρeff does not only affect the mean size of the size distributions, as it can also considerably affect the estimated absolute LDSAal concentration. With the Partector, the difference compared to the ELPI+ was also the highest in Prague, which is likely related to the suitable size range of the measurement (20–400 nm), as at least the result with the ELPI+ suggests a considerable contribution by particles larger than 400 nm on LDSAal. On the other hand, the result in Prague also suggests that the ELPI+ may have overestimated the contribution of particles larger than 400 nm, at least if compared to the SMPS. The Partector and ELPI+ seemed to agree with each other in terms of the LDSAal concentration when the accumulation mode of particles did not dominate the distribution (in Helsinki), whereas the DMPS and SMPS systematically measured lower concentrations than either ELPI+ or Partector. This difference in DMPS and SMPS compared to the electrical methods is likely related to fractal structure of particles (see Sect. 2.1.1). Also, the narrower measurement size ranges with the DMPS and SMPS may also explain some of the differences compared to the ELPI+, even though the measured size distributions suggest that only a small fraction of the larger particles were undetected with the DMPS or SMPS.
3.2.2 Effective density correction
The ρeff-corrected average LDSAal size distributions and concentrations are shown in Fig. 2. It should be noted that the ρeff correction was done only for the size distribution methods, as it is not possible to correct the Partector data. In Fig. 2, it can be observed that the differences with both size distributions and absolute concentrations decreased after the ρeff correction, which supports the idea that the differences in Fig. 1 were considerably related to the standard effective density approximation.
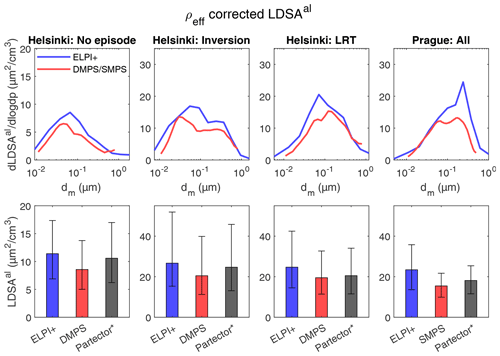
Figure 2Average LDSAal size distributions and concentrations measured with the different methods during the studied periods with corrections for the estimated particle effective density (1.1, 1.3, 1.7 and 2.0 g cm−3, respectively). The whiskers indicate the 25th and 75th percentiles of the measured concentrations. * Note that the particle effective density cannot be considered with the Partector. LDSAal data are shown in Table S2 (histograms in Figs. S12–S15). Note the different y-axis range for Helsinki: No episode.
The average LDSAal concentrations with the general assumptions compared to the ρeff-corrected ones with the size distribution methods are shown in Fig. 3. With the ELPI+, the standard ρeff assumption led to 16 % higher LDSAal concentration compared to the ρeff-corrected one in Prague, whereas, in Helsinki, the difference was <10 %. With the DMPS and SMPS, the standard ρeff assumption led to underestimated LDSAal values compared to the ρeff-corrected one, even though the difference was 5 % or less with all the cases. The differences in the average LDSAal concentrations between the methods with both the general assumptions and ρeff correction are shown in Fig. 4. After the ρeff correction, LDSAal with the DMPS and SMPS was 66 %–79 % of the ones measured with the ELPI+. The difference between Partector and ELPI+ still increased as the contribution of the accumulation mode increased, similar to the general assumption, but the difference was less than 23 % in all the environments (<18 % in Helsinki). Also, the differences in the scatter plot analysis with the hourly averaged data mainly decreased after the correction (Figs. S16–S18). Thus, the ρeff correction clearly decreased the differences between the methods, but it did not correct all the differences, and in particular, the absolute measured LDSAal concentrations can still be considerably different.
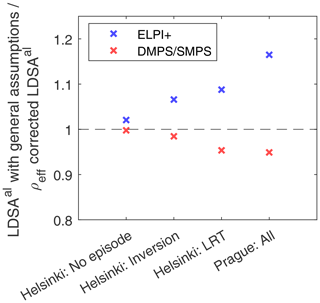
Figure 3Ratio between the measured LDSAal concentrations with and without correction for the particle effective density with the ELPI+, DMPS (Helsinki) or SMPS (Prague). See Table S2 for the measured average LDSAal concentrations (histograms in Figs. S12–S15).
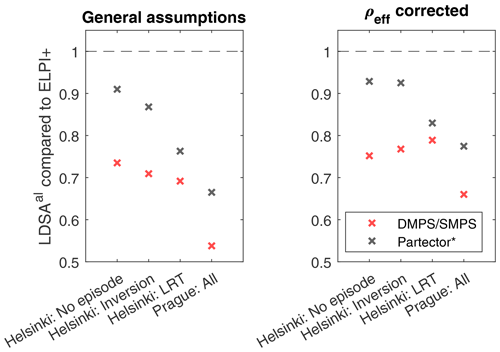
Figure 4Differences in the LDSAal concentrations measured with the DMPS (Helsinki), SMPS (Prague) and Partector compared to ELPI+ with and without correction for the particle effective density. * Note that the particle effective density cannot be considered in the Partector results. See Table S2 for the measured average LDSAal concentrations (histograms in Figs. S12–S15).
In terms of the operation principles of the devices, the results indicate that the ELPI+ is the most vulnerable to errors related to the wrongly assumed effective density in ambient conditions. This result can be explained in terms of the LDSAal size distributions with the size classification method of the ELPI+, which is dependent on the aerodynamic size and which is the key parameter only for particles roughly larger than 500 nm in the particle lung deposition. The overestimation of the total LDSAal concentration of the ELPI+ with the standard ρeff assumption (Fig. 3) can be explained with the conversion from the electric current to LDSAal, as the calculation considers the particles to have a larger mobility equivalent size than they have in reality, causing the conversion factors to LDSAal to be too high (see Lepistö et al., 2020). As seen, the majority of the LDSAal concentration in the studied sites was attributable to particles smaller than 500 nm (mobility equivalent diameter). The DMPS, SMPS and Partector are less vulnerable to errors related to the effective density if the concentration of particles larger than 500 nm is not high, as both the measurement (charging efficiency and size classification) and lung deposition efficiency are dependent on the mobility equivalent size. The slight underestimation in Fig. 3 is related to the concentrations of particles larger than about 500 nm, where the dominant deposition method changes from diffusion to impaction, causing the DMPS and SMPS to underestimate the deposition efficiency.
But, as mentioned, the ρeff correction did not fix all the limitations with the measurement methods. Still, the limited effective size range of 20–400 nm with the Partector can cause considerable uncertainty with ambient aerosol, especially in regions with high PM2.5. Also, the DMPS and SMPS seemed to underestimate the absolute LDSAal concentration by roughly 5 %–25 % after the ρeff correction as well, compared to the electrical methods (Fig. 4). This result agrees with a study by Chang et al. (2022), where LDSAal measurements of a NSAM and SMPS were compared in Taipei. On the other hand, Chen et al. (2023) reported over 50 % differences between NSAM and SMPS in Taipei. The lower concentrations of DMPS and SMPS compared to electric methods can be explained with the measurement principles as the electric current after diffusion charger is dependent on the particle shape, whereas with the DMPS and SMPS, only the number of particles (assumed spherical) is measured. Thus, agglomerated structures, especially with larger particles, can cause variation in the methods (Sect. 2.1.1). It is worth noting that even though the effective density can be taken into account with the size distribution methods, the size- and time-dependence of ρeff is practically challenging to consider, especially in typical monitoring measurements similar to this study. Thus, even after an effective density correction, some instrument-dependent uncertainties related to the effective density remain in the measurement, which should be recognised when reporting LDSAal results. On the other hand, with DMPS and SMPS, the approximation of one averaged effective density for all the particles does not cause considerable uncertainties in the results due to the fact that both the measurement method and the lung deposition are mainly dependent on the mobility equivalent size of particles. This is demonstrated in Fig. S19, where example comparisons of DMPS and SMPS data with averaged, standard and size-dependent effective densities in Helsinki and Prague are shown. However, with the ELPI+, the operation principle does not fundamentally enable the utilisation of a size-dependent effective density (see Sect. 2.3), which should be acknowledged. Still, this uncertainty in ELPI+ can be estimated by comparing the density-corrected results to the DMPS/SMPS results which are not as vulnerable to errors in terms of varying effective density.
3.2.3 Effect of particle hygroscopicity
In Fig. 5, the average LDSAal size distributions with corrections for both the particle effective density and the hygroscopic growth are compared to the ones without any corrections and with corrections only for the effective density. As seen, the hygroscopicity had a strong effect on the LDSAal size distributions, especially with particles larger than 400 nm. In general, hygroscopicity correction decreased the lung deposition efficiency of particles smaller than 200 nm, whereas it increased the deposition efficiency of particles larger than 200–400 nm (see also Fig. S7). Quite surprisingly, with the ELPI+, ρeff-corrected and hygroscopicity-corrected LDSAal size distributions were rather close to the ones with the general assumptions. This result can be explained by the fact that the estimated LDSAal of particles larger than 400 nm decreased after the ρeff correction, whereas the hygroscopicity correction increased the estimated lung deposition of these larger particles. With the SMPS and DMPS, this similar behaviour did not occur, as the ρeff correction did not dramatically change the measured size distribution. Hence, with the SMPS and DMPS, the hygroscopicity-corrected size distributions varied more compared to the ones with the general assumptions. In general, after the hygroscopicity correction, the shapes of the size distributions with both methods agreed reasonably well with each other.
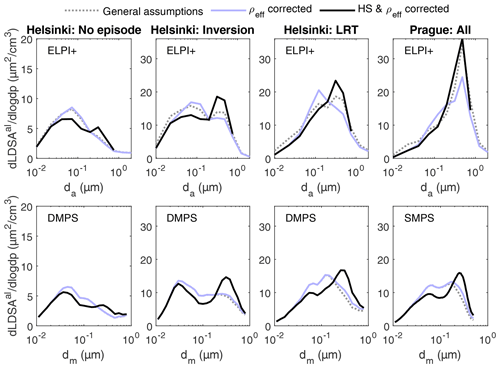
Figure 5Measured average LDSAal size distributions without corrections for the particle effective density and hygroscopic growth (from Fig. 1), with corrections for the effective density (from Fig. 2) and with corrections for both the effective density and the hygroscopic growth.
Even though the hygroscopicity correction can considerably change the estimated LDSAal size distributions, the effect on the measured absolute LDSAal concentration was less significant, which can be seen in Fig. 6. Note that the correction was not done for the Partector data. With the ELPI+, the LDSAal concentration with the general assumptions was 107 %–114 % of the hygroscopicity-corrected result in all the cases. With the DMPS and SMPS, the LDSAal with general assumptions was 95 %–104 % of the ones with the hygroscopicity correction. This result can be explained due to the balancing effects of particles smaller than 200 nm and larger than 200–400 nm in terms of the hygroscopicity correction. Thus, by coincidence, the accuracy of the absolute LDSAal concentration measurement was not significantly affected due to the particle hygroscopicity, which can also be seen in the hourly averaged scatter plots (Figs. S16–S18). However, it is worth noting that this result may depend on the location and urban environment. For example, the high concentration of accumulation mode particles can potentially cause an underestimation of LDSAal without a hygroscopicity correction. Also, it is important to note that the hygroscopicity correction still affected the relationship between the studied instruments (Fig. 6). In Helsinki, the DMPS and Partector measured 78 %–83 % and 87 %–103 % of the LDSAal measured with the ELPI+ after the hygroscopicity corrections, respectively. However, in Prague, the ratios dropped to 61 % and 73 % compared to the ELPI+, respectively. Thus, the uncertainty related to the particle hygroscopicity increased as the concentrations of the accumulation mode particles increased, similar to the particle effective density.
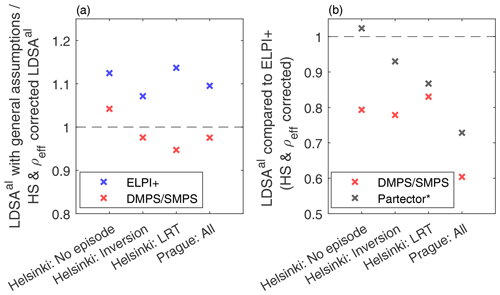
Figure 6(a) Ratio between the measured LDSAal concentrations with and without corrections for the particle effective density and hygroscopic growth. (b) Differences in the LDSAal concentrations measured with DMPS, SMPS and Partector compared to ELPI+, with corrections for the particle effective density and hygroscopicity. * Note that the corrections cannot be done for the Partector results. See Table S2 for the measured average LDSAal concentrations (histograms in Figs. S12–S15).
3.2.4 Location-dependence with the electrical particle sensors
As the electrical particle sensors are at least the most affordable solution to monitor LDSAal concentrations, it should be noted that in addition to the effective density and hygroscopicity, varying particles sizes also affect the performance of the sensors, as can also be seen with the Prague data, where the contribution of particles larger than 400 nm was underestimated with the Partector. However, varying particle size distributions within the suitable 20–400 nm size range can also affect the accuracy of the measurement, as the response between the diffusion-charged current and LDSAal is not linear (e.g. Todea et al., 2015), and the particle size distributions can vary considerably, depending on the nearby emission sources (e.g. Masiol et al., 2017; Harni et al., 2022; Lepistö et al., 2023). In Fig. 7, the average LDSAal concentrations with the ELPI+ and Partector from the measurements in Helsinki and Prague, as well as in Tampere and Düsseldorf, are compared. The data in Fig. 7 were not corrected based on the effective density and the hygroscopicity as data were not available for these corrections in the Tampere and Düsseldorf measurements.
In Fig. 7, it can be seen that the devices agreed rather well with each other in locations with high PN concentrations, whereas the difference increased in locations with low PN and high PM2.5. The difference with high PM2.5 can be explained with the contribution of accumulation mode particles larger than 400 nm, which typically increase as a function of PM2.5, and hence, the Partector underestimates the absolute LDSAal concentration. On the other hand, the ELPI+ may overestimate the contribution of accumulation mode particles without correction for the effective density, as seen in Figs. 1–3, but this overestimation alone does not explain the whole differences in Fig. 7. The high PN concentration indicates the contribution of ultrafine particles, which can be efficiently measured with the Partector, explaining why the differences decreased with higher PN concentrations. It is also worth noting that the performance of the Partector was good (> 80 % of ELPI+) in all the studied locations with high PN, including road traffic sites, airports and effects of residential wood combustion (see Table S3). Therefore, it seems that the varying particle sizes of the nearby emission sources do not dramatically affect the accuracy of the sensor measurement. For example, in the airport, LDSAal can be significantly contributed to by < 50 nm particles, whereas, in road traffic sites, the peak of the LDSAal size distribution is around 100 nm (Lepistö et al., 2023). On the other hand, in all the studied sites, the Partector measured lower concentrations than the ELPI+ (also after density corrections in Helsinki and Prague), suggesting a systematic difference between the methods, which may, e.g., be related to different lung deposition models in the device calibrations. Despite the underestimation of the Partector in sites with high PM2.5 and low PN, the correlation of the measured concentrations with the ELPI+ and Partector was still strong in all the studied sites (R2 is 0.85–0.98; Figs. S20–S22), showing that the challenges are mainly related to the utilised calibration factor.
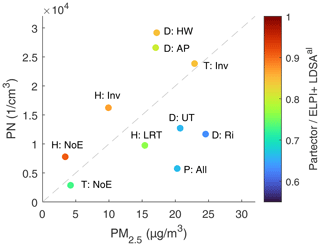
Figure 7Comparison of the LDSAal concentrations measured with the Partector and ELPI+ as a function of particle number (PN) and PM2.5 concentrations, which were calculated from the ELPI+ data. Each dot represents individual measurements in different locations (see Table S3). For measurements in Düsseldorf (D), the abbreviations are defined as follows: AP (airport), HW (highway), UT (urban traffic) and Ri (river). For measurements in Helsinki (H) and Tampere (T), the abbreviations are defined as follows: NoE (no episodes), Inv (Inversion) and LRT. All indicates the measurements in Prague (P).
The results in Fig. 7 show that the comparison of only electrical particle measurements of LDSAal can still be complicated due to the differences in the local and regional pollution levels. Therefore, a comparison of LDSAal measurements – even with the same device – can be challenging in different locations, depending on the regional pollution levels. On the other hand, it is worth discussing whether the LDSAal sensor measurement should be considered to represent only the LDSAal attributable to particles smaller than 400 nm, which would reduce this uncertainty. In this scenario, however, the particles larger than 400 nm should be removed from the sample, or the contribution of these particles should be estimated, e.g., by measuring the regional background concentration far away from any pollution sources. In Fig. S23, a similar comparison to the one in Fig. 7 between the ELPI+ and Partector was done by considering only particles smaller than 400 nm with the ELPI+. As a result, the Partector reported 5 %–29 % higher LDSAal concentrations than the ELPI+, which shows that the utilisation of the sensors only as an indication of the LDSAal concentration attributable to particles smaller than 400 nm is problematic if the contribution of larger particles is not considered.
3.2.5 Summary of the comparisons
As a summary, a comparison of the LDSAal results with different measurement methods can be complicated (see also Fig. S24). From a technological point of view, especially the particle size and effective density are important parameters when comparing the different methods. When considering the particle size, high concentrations of particles larger than 400 nm cause a significant underestimation of the absolute LDSAal concentration with the electrical particle sensors. The size distribution methods can consider varying particle sizes, but, on the other hand, the measurement size ranges can be different (similar to this study), which complicates the comparison. In this study, the uncertainty related to the varying measurement size ranges seemed, however, to be minimal. In addition, with the size distribution methods, especially the ELPI+ was vulnerable to errors related to the wrongly assumed particle effective density; the ELPI+ overestimated the LDSAal concentration by roughly up to 20 %, whereas, with the DMPS or SMPS, the uncertainty related to the effective density was <5 %, which is likely the case with the electrical particle sensors as well. However, when considering the conversion from the measured quantity to LDSAal, the DMPS and SMPS seemed to systematically underestimate the absolute LDSAal concentration by roughly 5 %–25 % compared to the electrical methods which likely better represent the actual surface area of particles (see also Chang et al., 2022; Chen et al., 2023). Thus, all the studied methods have both strengths and disadvantages in LDSAal measurement, and it is not possible to justifiably claim any of the methods to be the best method for the LDSAal measurement in general. Therefore, the disadvantages of each method should be carefully considered when reporting LDSAal results.
In addition, the effect of particle hygroscopicity should be recognised when reporting LDSAal results. Especially with the LDSAal size distributions, hygroscopic growth of particles can significantly change the result in ambient conditions. On the other hand, in terms of the absolute concentrations, neglected hygroscopicity did not considerably change the results due to the balancing effects with different particle sizes. Also, the measured LDSAal size distributions with the ELPI+ were surprisingly close without any corrections and with corrections for both the effective density and hygroscopicity, as the effective density and the hygroscopicity had balancing effects in the result. With the DMPS or SMPS, this similar phenomenon did not occur.
When considering the suitability of LDSAal in air quality monitoring measurements, it should be noted that the particle effective density and especially the hygroscopicity are practically challenging to consider. Thus, in comparison with other commonly utilised metrics (like PM2.5 or PN), there is considerably higher uncertainty with LDSAal, even if the measurements have been conducted with the same device. On the other hand, the challenges related to the LDSAal measurement seemed to become more relevant with the larger particles. For instance, the effective density of particles emitted from nearby local pollution sources is rather close to the standard. Also, the ultrafine or soot particles are typically hydrophobic, and the hygroscopic growth rates start to increase considerably with particles larger than 200–400 nm (Vu et al., 2015). In addition, the results in Fig. 7 and Table S3 show that electrical particle sensors are accurate in various urban environments despite different particle size distributions, as long as the particles are mainly smaller than 400 nm. In terms of particle health effects, the relevance of surface area is likely the highest with the smaller ultrafine and soot particles (Oberdörster et al., 2005; Schmid and Stoger, 2016; Hakkarainen et al., 2022), whereas with larger particles, secondary aerosol and soluble particles, the health effects have been strongly associated with the mass concentration (e.g. Lakey et al., 2016; Lin et al., 2016; Yu et al., 2022; Yang et al., 2023). Therefore, it is uncertain whether the surface area deposition is relevant in terms of larger and soluble particles. Thus, in terms of monitoring the effects of nearby local pollution sources in a dense air quality monitoring network, LDSAal should be a suitable and potential metric in terms of the particle health effects. This idea is supported by Figs. S24–S25, where the different methods agreed reasonably well in terms of LDSAal attributable to particles smaller than 400 nm, and the effective density or hygroscopicity corrections did not considerably change the result. Still, it should be acknowledged that, especially with the electrical sensors, particles larger than 400 nm are still measured and, therefore, can affect the accuracy of the measurement. Thus, with the electrical sensors, it would be reasonable to remove the larger particles from the sample or to utilise regional background measurements of LDSAal to reduce the uncertainty related to larger accumulation mode particles in the result.
The main strength of this study is that it provides comprehensive information of the differences between different LDSAal measurement methods in ambient measurements which have not been typically considered in previous studies. Therefore, the results help the interpretation of previous and future LDSAal studies conducted with different instrumentation. However, the uncertainties related to the analysis of this study, e.g. related to the determination of the particle effective density and hygroscopic growth, should be acknowledged. In this study, it was possible to estimate the average effective density of particles by comparing the ELPI+ and DMPS/SMPS size distributions, as well as the effects of hygroscopicity, based on a review by Vu et al. (2015). However, these parameters have spatiotemporal variability, and they depend on the particle size and composition. In general, these factors are challenging to determine (like ρeff in Prague), especially when considering the typical air quality monitoring measurements. Hence, not all the effects of particle effective density or hygroscopicity were recognised in the analysis, and thus the results of these parameters should be considered to be indicative. On the other hand, with the DMPS and SMPS, the uncertainty due to the average density assumption is less significant (Fig. S19), and the uncertainty in ELPI+ can be estimated by comparing the results to the DMPS and SMPS size distributions. Also, the analysis agrees with or is based on the existing literature, and therefore, the analysis can be considered to be reasonable. It is important to note that, according to the authors' knowledge, the effects of these parameters have not been previously analysed in terms of ambient LDSAal measurements. Hence, the results provide valuable information about these effects on the different LDSAal measurement methods in ambient conditions, even though the analysis includes necessary approximations. The same principle applies to the results of this study also in general; all of the studied instruments had both strengths and weaknesses, and hence, it is not possible to justifiably claim any of the methods to be the best in terms of the LDSAal measurement. Still, the results clearly show how the operation principles of the devices or the varying particle characteristics can affect the reported results in varying ambient conditions, which is crucial when comparing the results of different studies.
Additionally, differences with the utilised particle lung deposition function in the LDSAal measurement should be acknowledged. The particle lung deposition can be estimated with different models, and for example, both the ICRP and the Multiple-Path Particle Dosimetry model (MPPD; Asgharian et al., 2001) have been frequently utilised in LDSAal studies (e.g. Chang et al., 2022; Teinilä et al., 2022; Liu et al., 2023; Chen et al., 2023). In addition, the chosen input parameters for the models, like the human anatomy and physical activity, affect the lung deposition estimations. Thus, different LDSAal measurement methods can have differences in the applied lung deposition functions. This uncertainty is also difficult to estimate as, e.g., the Partector and ELPI+ report on LDSAal based on their own calibration (Fierz et al., 2014; Lepistö et al., 2020), whereas with the SMPS and DMPS, the utilised model is chosen by the user (which can be done with the ELPI+ as well). On the other hand, the different models agree reasonably well with each other, especially in terms of the shape of the deposition curve (e.g. Hofmann, 2011). However, for example, in Fig. 7, the Partector systematically measured lower concentrations than ELPI+ in all the studied sites, which suggest that varying deposition models in the calibration of the devices could also have had an effect on the results. Still, the uncertainties related to the deposition models are likely considerably less significant than the studied effects of particle effective density and hygroscopicity. Still, it would be beneficial to determine the common practices for measuring LDSAal in general with regard to the utilised lung deposition function. Also, further studies on the effects of the chosen lung deposition model, and its parameters, in terms of the LDSAal measurement with different devices would be beneficial to better understand how well the current measurement methods represent actual lung deposition, in addition to the effects of effective density and hygroscopic growth.
In all, the results indicate that the utilisation of LDSAal as a monitored metric in air quality monitoring measurements is complicated but also holds potential. The results suggest that the main challenges of the measurement start to have a considerable effect on the results only with high concentrations of accumulation mode particles larger than 200–400 nm. Also, the relevance of the surface area in terms of the adverse health effects is not as evident anymore with larger and soluble particles when compared to solid ultrafine particles or soot. Therefore, LDSAal could be a suitable parameter for detecting the spatial differences in the particulate pollution within cities as the effects of nearby pollution sources, like traffic, are commonly observed with ultrafine and soot particles that are smaller than 200 nm. As the current scientific evidence highlights the need for dense air quality monitoring networks and the implementation of new parameters like PN and BC in the monitoring, the sensitive and reasonably accurate measurement of ultrafine and soot particles with LDSAal could provide a cost-efficient method for monitoring measurements, e.g. with the electrical particle sensors. The results of this study suggest that there should not be a significant dependence of the urban environment in terms of the performance of the electrical particle sensors, as long as the local pollution dominates the sample and the effects of the larger accumulation mode particles are taken into account in the analysis. In this study, the detailed comparison was, however, only done for road traffic environments in lowly or moderately polluted regions. Also, the measurements of this study were rather short and conducted only during certain seasons. Thus, there is still a need for studies of particle effective density, hygroscopic growth and particle size distributions, along with the LDSAal measurement in different urban environments and in highly polluted regions, including long-term data, to better understand the universal suitability and behaviour of the metric.
The results of this study show that the comparison of ambient LDSAal measurements with different instruments and in different locations can be complicated. The comparisons of this study included one electrical particle sensor (Partector) and two different size distribution approaches (ELPI+ and DMPS/SMPS). In particular, the particle size, effective density and hygroscopicity can considerably affect the LDSAal measurement, and the effects are not the same with different devices. On the other hand, when considering all the required parameters for the measurement, e.g. the particle effective density, the differences between the methods decreased considerably but not completely. However, the challenges of the measurement were mainly related to the accumulation mode particles larger than 200–400 nm for which the surface area may not be as relevant in terms of the adverse health effects as with smaller ultrafine particles or soot. Therefore, regardless of the method, LDSAal should be very suitable when considering its utilisation to detect the effects of nearby local pollution sources in dense air quality monitoring networks, as long as the effects of larger particles are addressed either by removing them from the sample or by also measuring the regional background concentration. Still, further research on the relevance of the surface area in terms of larger and soluble particles, as well as the determination of common practises for the LDSAal measurement related to the utilised deposition model and its parameters, is needed to better understand the relevance and improve the suitability of LDSAal in terms of air quality monitoring.
Data are available from Zenodo at https://doi.org/10.5281/zenodo.13757126 (Lepistö, 2024).
The supplement related to this article is available online at: https://doi.org/10.5194/ar-2-271-2024-supplement.
TL: conceptualisation, methodology, validation, formal analysis, investigation, data curation, writing (original draft and review and editing) and visualisation. HL: methodology, investigation, data curation and writing (review and editing). LS and VS: investigation, data curation and writing (review and editing). LMFB, JH and LM: investigation and writing (review and editing). JVN and JO: resources, data curation and writing (review and editing). KT: methodology and writing (review and editing). HEM: resources and writing (review and editing). SS: methodology, investigation, writing (review and editing), project administration and funding acquisition. HT: methodology, writing (review and editing), project administration and funding acquisition. MDM and TR: writing (review and editing), project administration and funding acquisition.
At least one of the (co-)authors is a member of the editorial board of Aerosol Research. The peer-review process was guided by an independent editor, and the authors also have no other competing interests to declare.
Publisher's note: Copernicus Publications remains neutral with regard to jurisdictional claims made in the text, published maps, institutional affiliations, or any other geographical representation in this paper. While Copernicus Publications makes every effort to include appropriate place names, the final responsibility lies with the authors.
This study is part of the AeroSurf project funded by the Research Council of Finland (grant no. 356752).
This work has received funding from the European Union's Horizon 2020 research and innovation programme under grant no. 814978 (TUBE Project: Transport-Derived Ultrafines and the Brain Effects).
The study has received funding from the BC Footprint (grant no. 530/31/2019) and Future Spaces (grant no. 33250/31/2020) projects, which are funded by Business Finland, participating companies and municipal actors.
This research has been supported by the Research Council of Finland (grant no. 356752), the European Union's Horizon 2020 (grant no. 814978), and Business Finland (grant nos. 530/31/2019 and 33250/31/2020).
This paper was edited by Andreas Held and reviewed by two anonymous referees.
Aguilera, I., Dratva, J., Caviezel, S., Burdet, L., de Groot, E., Ducret-Stich, R. E., Eeftens, M., Keidel, D., Meier, R., Perez, L., Rothe, T., Schaffner, E., Schmit-Trucksäss, A., Ming-Yi, T., Schindler, C., Künzli, N., and Probst-Hensch, N: Particulate matter and subclinical atherosclerosis: associations between different particle sizes and sources with carotid intimamedia thickness in the SAPALDIA study, Environ. Health Persp., 124, 1700–1706, https://doi.org/10.1289/EHP161, 2016.
Asgharian, B., Hofmann, W., and Bergmann, R.: Particle Deposition in a Multiple-Path Model of the Human Lung, Aerosol Sci. Tech., 34, 332–339, https://doi.org/10.1080/02786820119122, 2001.
Barreira, L. M. F., Helin, A., Aurela, M., Teinilä, K., Friman, M., Kangas, L., Niemi, J. V., Portin, H., Kousa, A., Pirjola, L., Rönkkö, T., Saarikoski, S., and Timonen, H.: In-depth characterization of submicron particulate matter inter-annual variations at a street canyon site in northern Europe, Atmos. Chem. Phys., 21, 6297–6314, https://doi.org/10.5194/acp-21-6297-2021, 2021.
Cha, Y. and Olofsson, U.: Effective density of airborne particles in a railway tunnel from field measurements of mobility and aerodynamic size distributions, Aerosol Sci. Tech., 52, 886–899, https://doi.org/10.1080/02786826.2018.1476750, 2018.
Chang, P.-K., Griffith, S. M., Chuang, H.-C., Chuang, K.-J., Wang, Y.-H., Chang, K.-E., and Hsiao, T.-C.: Particulate matter in a motorcycle-dominated urban area: Source apportionment and cancer risk of lung deposited surface area (LDSA) concentrations, J. Hazard. Mater., 427, 128188, https://doi.org/10.1016/j.jhazmat.2021.128188, 2022.
Chen, G., Canonaco, F., Tobler, A., Aas, W., Alastuey, A., Allan, J., Atabakhsh, S., Aurela, M., Baltensperger, U., Bougiatioti, A., De Brito, J. F., Ceburnis, D., Chazeau, B., Chebaicheb, H., Daellenbach, K. R., Ehn, M., El Haddad, I., Eleftheriadis, K., Favez, O., Flentje, H., Font, A., Fossum, K., Freney, E., Gini, M., Green, D. C., Heikkinen, L., Herrmann, H., Kalogridis, A.-C., Keernik, H., Lhotka, R., Lin, C., Lunder, C., Maasikmets, M., Manousakas, M. I., Marchand, N., Marin, C., Marmureanu, L., Mihalopoulos, N., Močnik, G., Nęcki, J., O'Dowd, C., Ovadnevaite, J., Peter, T., Petit, J. E., Pikridas, M., Platt, S. M., Pokorná, P., Poulain, L., Priestman, M., Riffault, V., Rinaldi, M., Różański, K., Schwarz, J., Sciare, J., Simon, L., Skiba, A., Slowik, J. G., Sosedova, Y., Stavroulas, I., Styszko, K., Teinemaa, E., Timonen, H., Tremper, A., Vasilescu, J., Via, M., Vodička, P., Wiedensohler, A., Zografou, O., Minguillón, M. C., and Prévôt, A. S. H: European aerosol phenomenology – 8: Harmonised source apportionment of organic aerosol using 22 Year-long ACSM/AMS datasets, Environ. Int., 166, 107325, https://doi.org/10.1016/j.envint.2022.107325, 2022.
Chen, T.-L., Lai, C.-H., Chen, Y.-C., Ho, Y.-H., Chen, A. Y., and Hsiao, T.-C.: Source-oriented risk and lung-deposited surface area (LDSA) of ultrafine particles in a Southeast Asia urban area, Sci. Total Environ., 870, 161733, https://doi.org/10.1016/j.scitotenv.2023.161733, 2023.
Daellenbach, K. R., Uzu, G., Jiang, J., Cassagnes, L.-E., Leni, Z., Vlachou, A., Stefenelli, G., Canonaco, F., Weber, S., Segers, A., Kuenen, J. J. P., Schaap, M., Favez, O., Albinet, A., Aksoyoglu, S., Dommen, J., Baltensperger, U., Geiser, M., El Haddad, I., Jaffrezo J.-L., and Prévôt, A. S. H.: Sources of particulate-matter air pollution and its oxidative potential in Europe, Nature, 587, 414–419, https://doi.org/10.1038/s41586-020-2902-8, 2020.
de Jesus, A. L., Mahmudur Rahman, M., Mazaheri, M., Thompson, H., Knibbs, L. D., Jeong, C., Evans, G., Nei, W., Ding, A., Qiao, L., Li, L., Portin, H., Niemi, J. V., Timonen, H., Luoma, K., Petäjä, T., Kulmala, M., Kowalski, M., Peters, A., Cyrys, J., Ferrero, L., Manigrasso, M., Avino, P., Buonano, G., Reche, C., Querol, X., Beddows, D., Harrison, R. M., Sowlat, M. H., Sioutas, C., and Morawska, L.: Ultrafine particles and PM2.5 in the air of cities around the world: Are they representative of each other?, Environ. Int., 129, 118–135, https://doi.org/10.1016/j.envint.2019.05.021, 2019.
Dhaniyala, S., Fierz, M., Keskinen, J., and Marjamäki, M.: Instruments Based on Electrical Detection of Aerosols, in: Aerosol Measurement: Principles, Techniques, and Applications, 3d edn., John Wiley & Sons, Inc., 393–416, https://doi.org/10.1002/9781118001684.ch18, 2011.
Dockery, D. W., Pope, C. A., Xu, X., Spengler, J. D., Ware, J. H., Fay, M. E., Ferris Jr., B. G., and Speizer, F. E.: An association between air pollution and mortality in six U.S. cities, New Engl. J. Med., 329, 1753–1759, https://doi.org/10.1056/NEJM199312093292401, 1993.
Drinovec, L., Močnik, G., Zotter, P., Prévôt, A. S. H., Ruckstuhl, C., Coz, E., Rupakheti, M., Sciare, J., Müller, T., Wiedensohler, A., and Hansen, A. D. A.: The “dual-spot” Aethalometer: an improved measurement of aerosol black carbon with real-time loading compensation, Atmos. Meas. Tech., 8, 1965–1979, https://doi.org/10.5194/amt-8-1965-2015, 2015.
Edebeli, J., Spirig, C., Fluck, S., Fierz, M., and Anet, J.: Spatiotemporal Heterogeneity of Lung-Deposited Surface Area in Zurich Switzerland: Lung-Deposited Surface Area as a New Routine Metric for Ambient Particle Monitoring, Int. J. Public Health, 68, 1605879, https://doi.org/10.3389/ijph.2023.1605879, 2023.
Fierz, M., Meier, D., Steigmeier, P., and Burtscher, H.: Aerosol measurement by induced currents, Aerosol Sci. Tech., 48, 350–357, https://doi.org/10.1080/02786826.2013.875981, 2014.
Fissan, H., Neumann, S., Trampe, A., Pui, D., and Shin, W.: Rationale and principle of an instrument measuring lung deposited nanoparticle surface area, J. Nanopart. Res., 9, 53–59, https://doi.org/10.1007/978-1-4020-5859-2_6, 2006.
Hakkarainen, H., Salo, L., Mikkonen, S., Saarikoski, S., Aurela, M., Teinilä, K., Ihalainen, M., Martikainen, S., Marjanen P., Lepistö, T., Kuittinen, N., Aakko-Saksa, P., Pfeiffer, T. V., Timonen, H., Rönkkö, T., and Jalava, P. I.: Black carbon toxicity dependence on particle coating: Measurements with a novel cell exposure method, Sci. Total Environ., 838, 156543, https://doi.org/10.1016/j.scitotenv.2022.156543, 2022.
Harni, S. D., Saarikoski, S., Kuula, J., Helin, A., Aurela, M., Niemi, J. V., Kousa, A., Rönkkö, T., and Timonen, H.: Effects of emission sources on the particle number size distribution of ambient air in the residential area, Atmos. Environ., 293, 119419, https://doi.org/10.1016/j.atmosenv.2022.119419, 2022.
Heusinkveld, H., Wahle, T., Campbell, A., Westerink, R. H. C., Tran, L., Johnston, H., Stone, V., Cassee, F. R., and Schins, R. P. F.: Neurodegenerative and neurological disorders by small inhaled particles, NeuroToxicology, 113, 94–106, https://doi.org/10.1016/j.neuro.2016.07.007, 2016.
Hinds, W. C.: Aerosol technology, properties, behaviour, and measurement of airborne particles, John Wiley & Sons Inc, New York, ISBN: 978-1-118-59197-0, 1999.
Hofmann, W.: Modelling inhaled particle deposition in the human lung – A review, J. Aerosol Sci., 42, 693–724, https://doi.org/10.1016/j.jaerosci.2011.05.007, 2011.
ICRP (International Commission on Radiological Protection): Human respiratory tract model for radiological protection, ICRP Publication 66, Vol. 24, 492 pp., 1994.
Järvinen, A., Aitomaa, M., Rostedt, A., Keskinen, J., and Yli-Ojanperä, J.: Calibration of the new electrical low pressure impactor (ELPI+), J. Aerosol Sci., 69, 150–159, https://doi.org/10.1016/j.jaerosci.2013.12.006, 2014.
Järvinen, A., Kuuluvainen, H., Niemi, J. V., Saari, S., Dal Maso, M., Pirjola, L., Hillamo, R., Janka, K., Keskinen, J., and Rönkkö, T.: Monitoring urban air quality with a diffusion charger based electrical particle sensor, Urban Climate, 14, 441–456, https://doi.org/10.1016/j.uclim.2014.10.002, 2015.
Janssen, N., Hoek, G., Simic-Lawson, M., Fischer, P., van Bree, L., ten Brink, H., Keuken, M., Atkinson, R. W., Anderson, H. R., Brunekreef, B., and Cassee, F. R.: Black Carbon as an Additional Indicator of the Adverse Health Effects of Airborne Particles Compared with PM10 and PM2.5, Environ. Health Persp., 119, 1691–1699, https://doi.org/10.1289/ehp.1003369, 2011.
Jia, Y.-Y., Wang, Q., and Liu, T.: Toxicity Research of PM2.5 Compositions In Vitro, Int. J. Env. Res. Pub. He., 14, 232, https://doi.org/10.3390/ijerph14030232, 2017.
Keskinen, J., Pietarinen, K., and Lehtimäki, M.: Electrical low pressure impactor, J. Aerosol Sci., 23, 353–360, https://doi.org/10.1016/0021-8502(92)90004-F, 1992.
Kuula, J., Kuuluvainen, H., Rönkkö, T., Niemi, J. V., Saukko, E., Portin, H., Aurela, M., Saarikoski, S., Rostedt, A., Hillamo, R., and Timonen, H.: Applicability of Optical and Diffusion Charging-Based Particulate Matter Sensors to Urban Air Quality Measurements, Aerosol Air Qual. Res., 19, 1024–1039, https://doi.org/10.4209/aaqr.2018.04.0143, 2019.
Kuula, J., Kuuluvainen, H., Niemi, J. V., Saukko, E., Portin, H., Kousa, A., Aurela, M., Rönkkö, T., and Timonen, H.: Long-term sensor measurements of lung deposited surface area of particulate matter emitted from local vehicular and residential wood combustion sources, Aerosol Sci. Tech., 54, 190–202, https://doi.org/10.1080/02786826.2019.1668909, 2020.
Lakey, P., Berkemeier, T., Tong, H., Arangio, A. M., Lucas, K., Pöschl, U., and Shiraiwa, M.: Chemical exposure-response relationship between air pollutants and reactive oxygen species in the human respiratory tract, Sci Rep.-UK, 6, 32916, https://doi.org/10.1038/srep32916, 2016.
Leavey, A., Fang, J., Sahu, M., and Biswas, P.: Comparison of Measured Particle Lung-Deposited Surface Area Concentrations by an Aerotrak 9000 Using Size Distribution Measurements for a Range of Combustion Aerosols, Aerosol Sci. Tech., 47, 966–978, https://doi.org/10.1080/02786826.2013.803018, 2013.
Lepistö, T.: Data related to manuscript: Comparison of size distribution and electrical particle sensor measurement methods for particle lung deposited surface area (LDSAal) in ambient measurements with varying conditions (accepted for publication in Aerosol Research), Zenodo [data set], https://doi.org/10.5281/zenodo.13757126, 2024.
Lepistö, T., Kuuluvainen, H., Juuti, P., Järvinen, A., Arffman, A., and Rönkkö, T.: Measurement of the human respiratory tract deposited surface area of particles with an electrical low pressure impactor, Aerosol Sci. Tech., 54, 958–971, https://doi.org/10.1080/02786826.2020.1745141, 2020.
Lepistö, T., Kuuluvainen, H., Lintusaari, H., Kuittinen, N., Salo, L., Helin, A., Niemi, J. V., Manninen, H. E., Timonen, H., Jalava, P., Saarikoski, S., and Rönkkö, T.: Connection between lung deposited surface area (LDSA) and black carbon (BC) concentrations in road traffic and harbour environments, Atmos. Environ., 272, 118931, https://doi.org/10.1016/j.atmosenv.2021.118931, 2022.
Lepistö, T., Lintusaari, H., Oudin, A., Barreira, L. M. F., Niemi, J. V., Karjalainen, P., Salo, L., Silvonen, V., Markkula, L., Hoivala, J., Marjanen, P., Martikainen, S., Aurela, M., Reyes Reyes, F., Oyola, P., Kuuluvainen, H., Manninen, H. E., Schins, R. P. F., Vojtisek-Lom, M., Ondracek, J., Topinka, J., Timonen, H., Jalava, P., Saarikoski, S., and Rönkkö, T.: Particle lung deposited surface area (LDSAal) size distributions in different urban environments and geographical regions: Towards understanding of the PM2.5 dose–response, Environ. Int., 180, 108224, https://doi.org/10.1016/j.envint.2023.108224, 2023.
Levy, M. E., Zhang, E., Khalizov, A. F., Zheng, J., Collins, D. R., Glen, C. R., Wang, Y., Yu, X.-Y., Luke, W., Jayne, J. T., and Olaguer, E.: Measurements of submicron aerosols in Houston, Texas during the 2009 SHARP field campaign, J. Geophys. Res.-Atmos., 118, 10518–10534, https://doi.org/10.1002/jgrd.50785, 2013.
Lin, H., Tao, J., Du, Y., Liu, T., Qian, Z., Tian, L., Di, Q., Rutherford, S., Guo, L., Zeng, W., Xiao, J., Li, X., He, Z., Xu, Y., and Ma, W.: Particle size and chemical constituents of ambient particulate pollution associated with cardiovascular mortality in Guangzhou, China, Environ. Pollut. B, 208, 758–766, https://doi.org/10.1016/j.envpol.2015.10.056, 2016.
Liu, X., Hadiatullah, H., Zhang, X., Trechera, P., Savadkoohi, M., Garcia-Marlès, M., Reche, C., Pérez, N., Beddows, D. C. S., Salma, I., Thén, W., Kalkavouras, P., Mihalopoulos, N., Hueglin, C., Green, D. C., Tremper, A. H., Chazeau, B., Gille, G., Marchand, N., Niemi, J. V., Manninen, H. E., Portin, H., Zikova, N., Ondracek, J., Norman, M., Gerwig, H., Bastian, S., Merkel, M., Weinhold, K., Casans, A., Casquero-Vera, J. A., Gómez-Moreno, F. J., Artíñano, B., Gini, M., Diapouli, E., Crumeyrolle, S., Riffault, V., Petit, J.-E., Favez, O., Putaud, J.-P., dos Santos, S. M., Timonen, H., Aalto, P. P., Hussein, T., Lampilahti, J., Hopke, P. K., Wiedensohler, A., Harrison, R. M., Petäjä, T., Pandolfi, M., Alastuey, A., and Querol, X.: Ambient air particulate total lung deposited surface area (LDSA) levels in urban Europe. Sci. Total Environ., 898, 165466, https://doi.org/10.1016/j.scitotenv.2023.165466, 2023.
Lizonova, D., Nagarkar, A., Demokritou, P., and Kelesidis, G.: Effective density of inhaled environmental and engineered nanoparticles and its impact on the lung deposition and dosimetry, Part. Fibre Toxicol., 21, 7, https://doi.org/10.1186/s12989-024-00567-9, 2024.
Löndahl, J., Möller, W., Pagels, J. H., Kreyling, W. G., Swietlicki, E., and Schmid, O.: Measurement Techniques for Respiratory Tract Deposition of Airborne Nanoparticles: A Critical Review, J. Aerosol Med. Pulm. D., 27, 229–254, https://doi.org/10.1089/jamp.2013.1044, 2014.
Lu, J., Shen, X., Ma, Q., Yu, A., Hu, X., Zhang, Y., Liu, Q., Liu, S., Che, H., Zhang, H., and Sun, J.: Size-resolved effective density of ambient aerosols measured by an AAC–SMPS tandem system in Beijing, Atmos. Environ., 318, 120226, https://doi.org/10.1016/j.atmosenv.2023.120226, 2024.
Marra, J., Voetz, M., and Kiesling, H. J.: Monitor for detecting and assessing exposure to airborne nanoparticles, J. Nanopart. Res., 12, 21–37, https://doi.org/10.1007/s11051-009-9695-x, 2019.
Masiol, M., Harrison, R. M., Vu, T. V., and Beddows, D. C. S.: Sources of sub-micrometre particles near a major international airport, Atmos. Chem. Phys., 17, 12379–12403, https://doi.org/10.5194/acp-17-12379-2017, 2017.
Oberdörster, G., Oberdörster, E., and Oberdörster, J.: Nanotoxicology: an emerging discipline evolving from studies of ultrafine particles, Environ. Health Persp., 113, 823–839, https://doi.org/10.1289/ehp.7339, 2005.
Ohlwein, S., Kappeler, R., Kutlar Joss, M., Künzli, N., and Hoffman, B.: Health effects of ultrafine particles: a systematic literature review update of epidemiological evidence, Int. J. Public Health, 64, 547–559, https://doi.org/10.1007/s00038-019-01202-7, 2019.
Onasch, T. B., Trimborn, A., Fortner, E. C., Jayne, J. T., Kok, G. L., Williams, L. R., Davidovits, P., and Worsnop, D. R.: Soot Particle Aerosol Mass Spectrometer: Development, Validation, and Initial Application, Aerosol Sci. Tech., 46, 804–817, https://doi.org/10.1080/02786826.2012.663948, 2012.
Patel, S., Leavey, A., Sheshadri, A., Kumar, P., Kandikuppa, S., Tarsi, J., Mukhopadhyay, K., Johnson, P., Balakrishnan, K., Schechtman, K. B., Castro, M., Yadama, G., and Biswas, P.: Associations between household air pollution and reduced lung function in women and children in rural southern India, J. Appl. Toxicol., 38, 1405–1415, https://doi.org/10.1002/jat.3659, 2018.
Park, M., Joo, H. S., Lee, K., Jang, M., Kim, S. D., Kim, I., Borlaza, L. J. S., Lim, H., Shin, H., Chung, K. H., Choi, Y.-H., Park, S. G., Bae, M.-S., Lee, J., Song, H., and Park, K.: Differential toxicities of fine particulate matters from various sources, Sci. Rep.-UK, 8, 17007, https://doi.org/10.1038/s41598-018-35398-0, 2018.
Pinault, L., Tjepkema, M., Crouse, D. L., Weichenthal, S., van Donkelaar, A., Martin, R. V., Brauer, M., Chen, H., and Burnett, R. T.: Risk estimates of mortality attributed to low concentrations of ambient fine particulate matter in the Canadian community health survey cohort, Environ. Health-UK, 15, 18, https://doi.org/10.1186/s12940-016-0111-6, 2016.
Reche, C., Viana, M., Brines, M., Pérez, N., Beddows, D., Alastuey, A., and Querol, X.: Determinants of aerosol lung-deposited surface area variation in an urban environment, Sci. Total Environ., 517, 38–47, https://doi.org/10.1016/j.scitotenv.2015.02.049, 2015.
Rissler, J., Nordin, E. Z., Eriksson, A. C., Nilsson, P. T., Frosch, M., Sporre, M. K., Wierzbicka, A., Svenningsson, B., Löndahl, J., Messing, M. E., Sjogren, S., Hemmingsen, J. G., Loft, S., Pagels, J. H., and Swietlicki, E.: Effective Density and Mixing State of Aerosol Particles in a Near-Traffic Urban Environment. Environ. Sci. Technol., 48, 6300–6308, https://doi.org/10.1021/es5000353, 2014.
Salo, L., Hyvärinen, A., Jalava, P., Teinilä, K., Hooda, R. K., Datta, A., Saarikoski, S., Lintusaari, H., Lepistö, T., Martikainen, S., Rostedt, A., Sharma, V. P., Rahman, M. H., Subudhi, S., Asmi, E., Niemi, J. V., Lihavainen, H., Lal, B., Keskinen, J., Kuuluvainen, H., Timonen, H., and Rönkkö, T.: The characteristics and size of lung-depositing particles vary significantly between high and low pollution traffic environments, Atmos. Environ., 255, 118421, https://doi.org/10.1016/j.atmosenv.2021.118421, 2021.
Schmid, I. and Stoeger, T.: Surface area is the biologically most effective dose metric for acute nanoparticle toxicity in the lung, J. Aerosol Sci., 99, 133–143, https://doi.org/10.1016/j.jaerosci.2015.12.006, 2016.
Segersson, D., Johansson, C., and Forsberg, B.: Near-Source Risk Functions for Particulate Matter Are Critical When Assessing the Health Benefits of Local Abatement Strategies, Int. J. Env. Res. Pub. He., 18, 6847, https://doi.org/10.3390/ijerph18136847, 2021.
Shin, W. G., Pui, D. Y. H., Fissan, H., Neumann, S., and Trampe, A.: Calibration and numerical simulation of Nanoparticle Surface Area Monitor (TSI Model 3550 NSAM), J. Nanopart. Res., 9, 61–69, https://doi.org/10.1007/s11051-006-9153-y, 2007.
Sidwell, A., Smith, S.C., and Roper, C.: A comparison of fine particulate matter (PM2.5) in vivo exposure studies incorporating chemical analysis, J. Toxicol. Env. Heal. B, 25, 422–444, https://doi.org/10.1080/10937404.2022.2142345, 2022.
Silvonen, V., Salo, L., Raunima, T., Vojtisek-Lom, M., Ondracek, J., Topinka, J., Schins, R.P.F., Lepistö, T., Lintusaari, H., Saarikoski, S., Barreira, L.M.F., Hoivala, J., Markkula, L., Kulmala, I., Vinha, J., Karjalainen, P., and Rönkkö, T.: Lung-depositing surface area (LDSA) of particles in office spaces around Europe: Size distributions, I/O-ratios and infiltration, Build. Environ., 246, 110999, https://doi.org/10.1016/j.buildenv.2023.110999, 2023.
Strak, M., Weinmayr, G., Rodopoulou, S., Chen, J., de Hoogh, K., Andersen, Z. J., Atkinson, R., Bauwelinck, M., Bekkevold, T., Bellander, T., Boutron-Ruault, M.-C., Brandt, J., Cesaroni, G., Concin, H., Fecht, D., Forastiere, F., Gulliver, J., Hertel, O., Hoffmann, B., Hvidtfeldt, U. A., Janssen, N. A. H., Jöckel, K.-H. Jørgensen, J. T., Ketzel, M., Klompmaker, J. O., Lager, A., Leander, K., Liu, S., Ljungman, P., Magnusson, P. K. E., Mehta, A. J., Nagel, G., Oftedal, B., Pershagen, G., Peters, A., Raaschou-Nielsen, O., Renzi, M., Rizzuto, D., van der Schouw, Y. T., Schramm, S., Severi, G., Sigsgaard, T., Sørensen, M., Stafoggia, M., Tjønneland, A., Verschuren, W. M. M., Vienneau, D., Wolf, K., Katsouyanni, K., Brunekreef, B., Hoek, G., and Samoli, E.: Long term exposure to low level air pollution and mortality in eight European cohorts within the ELAPSE project: pooled analysis, BMJ, 374, 1904, https://doi.org/10.1136/bmj.n1904, 2021.
Teinilä, K., Timonen, H., Aurela, M., Kuula, J., Rönkkö, T., Hellèn, H., Loukkola, K., Kousa, A., Niemi, J. V., and Saarikoski, S.: Characterization of particle sources and comparison of different particle metrics in an urban detached housing area, Finland, Atmos. Environ., 272, 118939, https://doi.org/10.1016/j.atmosenv.2022.118939, 2022.
Teinilä, K., Saarikoski, S., Lintusaari, H., Lepistö, T., Marjanen, P., Aurela, M., Hellén, H., Tykkä, T., Lampimäki, M., Lampilahti, J., Barreira, L., Mäkelä, T., Kangas, L., Hatakka, J., Harni, S., Kuula, J., Niemi, J. V., Portin, H., Yli-Ojanperä, J., Niemelä, V., Jäppi, M., Lehtipalo, K., Vanhanen, J., Pirjola, L., Manninen, H. E., Petäjä, T., Rönkkö, T., and Timonen, H.: Measurement report: Wintertime aerosol characterization at an urban traffic site in Helsinki Finland, Zenodo, https://doi.org/10.5281/zenodo.13254917, 2024.
Todea, A. M., Beckmann, S., Kaminski, H., and Asbach, C.: Accuracy of electrical aerosol sensors measuring lung deposited surface area concentrations, J. Aerosol Sci., 89, 96–109, https://doi.org/10.1016/j.jaerosci.2015.07.003, 2015.
Todea, A. M., Beckmann, S., Kaminski, H., Bard, D., Bau, S., Clavaguera, S., Dahmann, D., Dozol, H., Dziurowitz, N., Elihn, K., Fierz, M., Lidén, G., Meyer-Plath, A., Monz, C., Neumann, V., Pelzer, J., Simonow, B. K., Thali, P., Tuinman, I., van der Vleuten, A., Vroomen, H., and Asbach, C.: Intercomparison of personal monitors for nanoparticles exposure at workplaces and in the environment, Sci. Total Environ., 605–606, 929–945, https://doi.org/10.1016/j.scitotenv.2017.06.041, 2017.
Vallabani, N. V. S., Gruzieva, O., Elihn, K., Juárez-Facio, A. T., Steimer, S. S., Kuhn, J., Silvergren, S., Portugal, J., Piña, B., Olofsson, U., Johansson, C., and Karlsson, H. L.: Toxicity and health effects of ultrafine particles: Towards an understanding of the relative impacts of different transport modes, Environ. Res., 231, 116186, https://doi.org/10.1016/j.envres.2023.116186, 2023.
Virtanen, A., Rönkkö, T., Kannosto, J., Ristimäki, J., Mäkelä, J. M., Keskinen, J., Pakkanen, T., Hillamo, R., Pirjola, L., and Hämeri, K.: Winter and summer time size distributions and densities of traffic-related aerosol particles at a busy highway in Helsinki, Atmos. Chem. Phys., 6, 2411–2421, https://doi.org/10.5194/acp-6-2411-2006, 2006.
Vodonos, A., Awad, Y. A., and Schwartz, J.: The concentration-response between long-term PM2.5 exposure and mortality; A meta-regression approach, Environ. Res., 166, 677–689, https://doi.org/10.1016/j.envres.2018.06.021, 2018.
Vu, T. V., Delgado-Saborit, J. M., and Harrison, R. M.: A review of hygroscopic growth factors of submicron aerosols from different sources and its implication for calculation of lung deposition efficiency of ambient aerosols, Air Qual. Atmos. Hlth., 8, 429–440, https://doi.org/10.1007/s11869-015-0365-0, 2015.
WHO (World Health Organization): WHO global air quality guidelines: particulate matter (PM2.5 and PM10), ozone, nitrogen dioxide, sulfur dioxide and carbon monoxide, WHO, https://apps.who.int/iris/handle/10665/345329 (last access: 7 May 2024), 2021.
Wu, C.-A., Chen, Y.-T., Young, L.-H., Chang, P.-K., Chou, L.-T., Chen, A. Y., and Hsiao, T.-C.: Ultrafine particles in urban settings: A combined study of volatility and effective density revealed by VT-DMA-APM, Atmos. Environ., 312, 120054, https://doi.org/10.1016/j.atmosenv.2023.120054, 2023.
Yang, M., Zeng, H.-X., Wang, X.-F., Hakkarainen, H., Leskinen, A., Komppula, M., Roponen, M., Wu, Q.-Z., Xu, S.-L., Lin, L.-Z., Liu, R.-Q., Hu, L.-W. Yang, B.-Y., Zeng, X.-W., Dong, G.-H., and Jalava, P.: Sources, chemical components, and toxicological responses of size segregated urban air PM samples in high air pollution season in Guangzhou, China, Sci. Total Environ., 865, 161092, https://doi.org/10.1016/j.scitotenv.2022.161092, 2023.
Yin, Z., Ye, X., Jiang, S., Tao, Y., Shi, Y., Yang, X., and Chen, J.: Size-resolved effective density of urban aerosols in Shanghai, Atmos. Environ., 100, 133–140, https://doi.org/10.1016/j.atmosenv.2014.10.055, 2015.
Yu, W., Chen, J., Qin, W., Ahmad, M. Zhang, Y., Sun, Y., Xin, K., and Ai, J.: Oxidative potential associated with water-soluble components of PM2.5 in Beijing: The important role of anthropogenic organic aerosols, J. Hazard. Mater., 433, 128839, https://doi.org/10.1016/j.jhazmat.2022.128839, 2022.