the Creative Commons Attribution 4.0 License.
the Creative Commons Attribution 4.0 License.
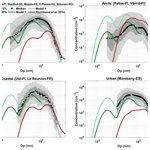
Parameterization of particle formation rates in distinct atmospheric environments
Tuomo Nieminen
Rima Baalbaki
Putian Zhou
Pauli Paasonen
Risto Makkonen
Martha Arbayani Zaidan
Nina Sarnela
Tuija Jokinen
Imre Salma
Máté Vörösmarty
Tuukka Petäjä
Veli-Matti Kerminen
Markku Kulmala
Atmospheric particle formation rate (J) is one of the key characteristics of new particle formation (NPF) processes worldwide. It is related to the development of ultrafine particle growth to cloud condensation nuclei (CCN) and, hence, Earth radiative forcing in global models, which helps us to better understand the impact of NPF on cloud properties and climate change. In this work, we parameterized four semi-empirical J models for 5 nm atmospheric particles using field measurements obtained from distinct environments that varied from clean to heavily polluted regions and from tropical to polar regions. The models rely primarily on sulfuric acid as a condensing vapor, a condensation sink to account for the vapor loss, and relative humidity for the meteorological contribution to J. However, the dependencies between J, condensation sink, and relative humidity are affected by their interlinked relations to sources and sinks of condensable vapors other than sulfuric acid and that of the potential traffic emissions to the observed size range. The parameterization results showed that our models were able to produce plausible predictions for boreal forest environments, heavily polluted environments, and biogenic environments with high relative humidity. We further tested the models in the global simulation module Tracer Model 5 (TM5, massively parallel version) to simulate the particle number size distribution across 14 global atmospheric measurement sites. The simulated results showed satisfactory predictions of particle number concentrations for all of the tested environments, with significant improvement in the nucleation mode and better prediction accuracy for the Aitken and accumulation modes compared to the binary sulfuric acid–organic vapor model in Riccobono et al. (2014). Our study has successfully provided powerful tools for predicting J5 on a global scale across various environment types using the most essential and more accessible variables involved in the NPF processes. Essentially, this work reinforces the necessity for global research into the investigation of environment-oriented meteorology-involved NPF processes.
- Article
(4973 KB) - Full-text XML
-
Supplement
(1167 KB) - BibTeX
- EndNote
Atmospheric new particle formation (NPF) is a natural phenomenon observed globally (Bousiotis et al., 2021; Brean et al., 2023; Gordon et al., 2017; Kerminen et al., 2018; Nieminen et al., 2018). As particles form and grow on regional scales, they can reach large enough sizes at which they can act as cloud condensation nuclei (CCN) for water vapor to condense onto when forming clouds. This process affects cloud properties (Roldin et al., 2011; Sanchez et al., 2016; Spracklen et al., 2008) and ultimately the global climate, depending on the particle numbers, their sizes, and their chemical compositions (Bellouin et al., 2020; Calvo et al., 2013; Uno et al., 2020). Particle formation rate (J) is an essential parameter describing the NPF intensity, which is often utilized to represent NPF in global models to simulate the effect of NPF on cloud properties and radiative forcing on Earth. To derive a representative parameterization of J for global simulation, we require a broad understanding of NPF in different environments, temporally and spatially. That being the case, it is essential to obtain the atmospheric measurements of both particle number size distributions and NPF precursor vapors for atmospheric observations and model developments.
The NPF processes have been investigated and parameterized based on particle formation mechanism theories (Chang et al., 2009; Kulmala et al., 2001; Lehtinen and Kulmala, 2003); field measurements from specific environments such as pristine boreal forests (Kulmala et al., 2001; Nieminen et al., 2011; Paasonen et al., 2010), urban cities (Salma et al., 2011, 2016, 2021; Salma and Németh, 2019; Zhang et al., 2010), rural areas (Lee et al., 2019; Yli-Juuti et al., 2009), and marine environments (Zhang et al., 2010); and chamber experiments (Kirkby et al., 2011; Lehtipalo et al., 2018). In addition to neutral particle formation mechanisms, the ion-induced nucleation is also covered in J parameterizations (e.g., Nieminen et al., 2011; Määttänen et al., 2018). The existing literature primarily focused on the activation and survival of nucleation mode particles down to 1.5 nm, involving complex microphysics of aerosol particles, such as nanometer cluster production and losses due to cluster coagulation and growth (Bousiotis et al., 2021; Chu et al., 2019; Kerminen et al., 2018; Nieminen et al., 2018). Furthermore, distinct effects on particle formation rates influenced by the same factors were seen in comparable environmental settings. However, these environment-specific models typically have limited applications for global simulation implementations that encompass the diverse atmospheric conditions on Earth.
To simulate particle formation rates, one usually starts from the nucleation mode size range. Global modelers have been facing great challenges in simulating nucleation mode particles because large-scale models have limited capabilities in treating the complicated aerosol dynamics taking place in the sub-5 nm particle size range. The formation rate at 5 nm is shown to be important because, after sizes of about a few nanometers in diameter, particle growth rates show relatively limited variability in different environments (Kulmala et al., 2022a, b, 2023). In addition, we currently do not have a good enough theoretical understanding of the processes dictating particle growth rates at the smallest sizes or the survival of such particles from coagulation scavenging (e.g., Cai et al., 2022; Tuovinen et al., 2022; Marten et al., 2022).
The essential parameters for J parameterizations should include at least one type of precursor vapor, and some may also cover meteorological parameters and the sinks for vapors and particles. For instance, sulfuric acid (H2SO4), as the most known precursor vapor, plays a critical role in particle formation and growth processes due to its low volatility (Kulmala et al., 2004; Myllys et al., 2019). In the earlier parameterizations of NPF mechanisms, J correlated (linearly or squared) with H2SO4 concentrations in various environments (Paasonen et al., 2010). In terms of meteorology, air temperature (T), relative humidity (RH), global solar radiation (GRad), wind speed (WS), and wind direction influence the particle formation rates in certain environments as well (Laarne et al., 2022; Salma et al., 2021; Zaidan et al., 2018). The variation of T can influence the precursor vapor formation and the stability of NPF processes: a higher T can enhance the biogenic emissions that participate in particle formation in a boreal forest (Dada et al., 2017; Nieminen et al., 2015), while a lower T favored H2SO4–amine cluster stability in a megacity (Deng et al., 2020). RH can impact the precursor vapor formations as well as the aerosol formation rates (Ding et al., 2021; Hellmuth, 2006). The variation of RH is dependent on T, so that the increase in T during daytime increases the planetary boundary layer height (PBLH), which in turn dilutes the air mixture and decreases the RH (Liu et al., 2018) as well as particle number concentrations (Mazon et al., 2016) in the atmosphere. For condensable vapor loss, we usually include the term “condensation sink” (CS), which describes the loss rate of condensable vapors to aerosol particles, and it typically declines before an NPF event starts. H2SO4 concentrations, on the other hand, increase due to the reduction in CS, which means that the condensable vapors are not lost onto the aerosol particles as efficiently as they would be at greater CS values (Hellmuth, 2006; Kulmala et al., 2012).
In general, the developed J models underestimate the observed particle number concentration, which may be due to NPF schemes being poorly represented in these models. Many J parameterization works were conducted, focusing on the formation mechanisms from sulfuric acid (Paasonen et al., 2010), sulfuric acid–water (Määttänen et al., 2018), sulfuric acid–ammonia (Glasoe et al., 2015), and sulfuric acid–organic vapor (Paasonen et al., 2010; Riccobono et al., 2014). However, some models are likely only applicable to certain types of environment or primarily cover the microphysics of the particle nucleation in the sub-3 nm range, where the nucleated clusters face higher instability due to the higher evaporation rates than condensation rates (Deng et al., 2021; Wang et al., 2011). Bergman et al. (2022) attempted an organic-vapor-based NPF scheme in addition to the commonly used binary water–sulfuric-acid-based scheme to simulate global particle formation and number concentrations. This scheme improved the simulated number concentrations across the observation stations, although they were still underestimated compared to the observations, suggesting that the parameterization of early growth of particles to a diameter of 5 nm still requires improvement.
To predict the particle formation rate at 5 nm originating from NPF and subsequent growth and to understand and predict the climatic impacts of NPF and initial growth on a global scale, we parameterize particle formation rates (J) at 5 nm using combined measurement data from six different environments: Hyytiälä (boreal forest close to a rural environment, Finland), Beijing (megacity, China), Värriö (remote boreal forest, Finland), Budapest (urban, Hungary), Agia Marina Xyliatos (rural, Cyprus), and Manacapuru (Amazonian basin, Brazil). The parameterizations of J were based on the analysis of atmospheric particle number–size distributions. Sulfuric acid concentrations, RH, and CS are the main input variables in the parameterization models. By including information from various types of environments, we will be able to demonstrate whether our models can adequately explain the formation rate of 5 nm particles on a wider environmental scale. The parameterized models are then incorporated into European Community Earth-System (EC-Earth) models to simulate particle formation rates on the global scale (EC-Earth, chemistry transport model TM5: Tracer Model 5, version TM5-chem-v3.0; details can be found in the Supplement) (Huijnen et al., 2010).
This work aims to provide an effective tool for global particle formation rate estimations. Our parameterizations have three main features: (1) the number of inputs is limited to the most essential parameters involved in the NPF process, (2) they do not involve complex microphysics at particles smaller than 5 nm, and (3) they cover a wide range of environment types. These features will enhance the applicability of the parameterizations for the purpose of global model application.
This study includes measurements from six different sites representing different environmental conditions. A summary of all of the locations and instrumentation used is given in Table S1 in the Supplement. Figure 1 shows the map of the measurement sites included in this study.
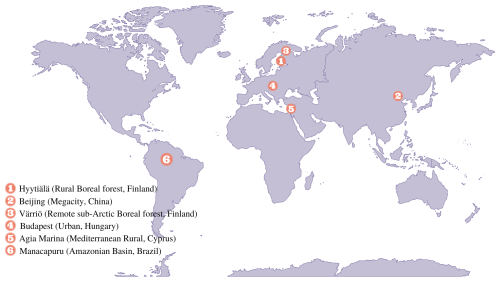
Figure 1Map of the measurement locations included in this study. The number markings indicate the exact locations of the measurements. Created using a template from Canva (https://www.canva.com/, last access: 8 May 2024).
2.1 Measurement sites
2.1.1 Rural boreal forest environment: Hyytiälä, Finland
The measurement data were obtained from the SMEAR II (Station for Measuring Ecosystem–Atmosphere Relations) station situated in a Scots pine (Pinus sylvestris) forest in Hyytiälä (61.1° N, 24.17° E; 181 m a.s.l.; Hari and Kulmala, 2005), southern Finland. This measurement site is described as having a rural regional background with minimal anthropogenic emissions. Hyytiälä data cover the period from 21 March 2016 to 18 August 2019.
2.1.2 Remote sub-arctic boreal forest: Värriö, Finland
The SMEAR I measurement station (67°45′ N, 29°36′ E; 390 m a.s.l.) is located on the top of Kotovaara hill in northeastern Finland. Similar to Hyytiälä, the site is also a rural background covered mainly by a Scots pine (Pinus sylvestris) forest located on the northern side of the Värriö fell range. However, it is affected by a potentially polluted air mass that comes from the Kola Peninsula rather than local industrial pollutants. A detailed description of the SMEAR I station can be found in Kyrö et al. (2014). The data used from Värriö are from 5 April to 13 August 2019.
2.1.3 Polluted megacity: Beijing, China
In Beijing, the measurements were performed on the western campus of the Beijing University of Chemical Technology (BUCT, 39.94° N, 116.30° E; 20 m a.s.l.). The sampling took place from outside the window on the fifth floor of the university building close to a street with busy traffic. For more details on the description of the BUCT measurement site, see Liu et al. (2020). The data are available from 29 May 2018 to 3 April 2019.
2.1.4 Urban site: Budapest, Hungary
The measurements took place at the Budapest platform of the Aerosol Research and Training (BpART) Laboratory (47.47° N, 19.06° E; 115 m a.s.l.) of Eötvös Loránd University situated on the bank of the Danube River. The site represents a mixed average atmosphere of the city center (Salma et al., 2016). The data are obtained from 22 March to 17 April 2018.
2.1.5 Mediterranean rural site: Agia Marina, Cyprus
The measurements were conducted at the Agia Marina Xyliatou (AMX) station (35.03° N, 33.05° E; 532 m a.s.l.) of the Cyprus Atmospheric Observatory (CAO). The site represents a rural background location situated at the foothills of the Troodos mountains, with agricultural land in the vicinity. The data are obtained between 22 February and 3 March 2018. For more details about the site, see, e.g., Baalbaki et al. (2021).
2.1.6 Amazonian basin: Manacapuru, Brazil
The Manacupuru measurement site is in a pastureland 70 km west of Manaus, Brazil, in central Amazonia. This site receives air masses from various resources, including rural, biogenic, and anthropogenic, from the nearby municipality (Manaus). The trace gases and meteorological measurements were performed during the GoAmazon2014/5 campaign at the T3 site (3.21° S, 60.6° W; 50 m a.s.l.), 10 km northeast of Manacapuru, Brazil (Martin et al., 2016; Schiro et al., 2018). A more detailed description of the measurement site can be found in Myers et al. (2022). The data cover the time period from 22 August to 9 October 2014.
2.2 Instrumentation
2.2.1 Sulfuric acid measurements and proxies
H2SO4 concentrations were measured at all of the sites, except for the Amazonian basin, using a chemical ionization atmospheric pressure interface time-of-flight (CI-APi-ToF) spectrometer (Eisele and Tanner, 1993; Jokinen et al., 2012) with as the reagent ion and analyzed using the tofTools package based on the MATLAB software (Junninen et al., 2010). In the Amazonian basin, H2SO4 concentrations were measured using a selected ion chemical ionization mass spectrometer (SICIMS); see Myers et al. (2022) for more details. The H2SO4 concentration measurements were taken from different levels, ranging from ground level up to 35 m above ground level (a.g.l.). The CI-APi-ToF spectrometers were calibrated uniformly before the measurement in each location following the technique described by Kürten et al. (2012), except for the Amazonian basin, where the SICIMS was calibrated following the scheme described in Mauldin et al. (1998).
To increase the applicability of our derived parameterization, H2SO4 proxy data from Hyytiälä and Beijing were included as an additional testing dataset. The proxy data were calculated using the proxy specific to the boreal forest environment and the polluted megacity developed by Dada et al. (2020). For Hyytiälä, the sulfuric acid proxy data range from 22 August to 25 December 2016 and from 8 March 2018 to 26 February 2019, denoted as HyytiäläSAprx. For Beijing, the time period is from 15 March to 3 April 2019, denoted as BeijingSAprx. The subscript “SAprx” (SA as in sulfuric acid) in HyytiäläSAprx and BeijingSAprx indicates that the datasets utilize the H2SO4 concentration from proxies as input for the testing dataset.
2.2.2 Particle number size distribution
The particle number size distribution (PNSD) measurements were obtained from different types of setups at each site. Hyytiälä had twin differential mobility particle sizers (DMPSs; Aalto et al., 2001). Värriö had DMPSs (Jokinen et al., 2022). Beijing had a particle size distribution (PSD) system with a nano-differential mobility analyzer (DMA) and an aerodynamic particle sizer (APS) (Zhou et al., 2021). Budapest had a flow-switching-type DMPS (6–1000 nm; Salma et al., 2016). Cyprus had a neutral cluster and air ion spectrometer (NAIS) and a scanning mobility particle sizer (SMPS; Baalbaki et al., 2021). In the Amazonian basin, the measurements were conducted using a SMPS (10–1000 nm). It is important to note that we do not aim to compare the PNSD measurements from all of the chosen sites. Instead, the PNSD measurements were used to calculate the formation rates based on changes in particle number concentrations under local conditions.
2.2.3 Meteorological variable
The meteorological variables included in this study are RH (%) and T (°C). In Hyytiälä, RH and T were measured at 16.8 m using a Rotronic MP102H RH sensor (Rotronic Hygromet MP102H with Hygroclip HC2–S3, Rotronic AG, Bassersdorf, Switzerland). In Värriö, RH and T were measured by a Rotronic MP106A captive sensor. In Beijing, RH and T were monitored by the Vaisala weather station (AWS310). In Budapest, RH and T were monitored using a Vaisala HMP45D temperature and humidity probe and a Vaisala WAV15A anemometer located at the site of the BpART laboratory. In Cyprus, RH and T were measured by a meteorological station in a nearby village (35.01° N, 33.05° E), 2.85 km away from the measurement site. In the Amazonian basin, RH and T were measured at the Atmospheric Radiation Measurement (ARM) user facility.
2.3 Data analysis
2.3.1 Calculation of particle formation rates
To develop more inclusive and generalized models, the parameterization included data from both NPF event days and non-NPF-event days. This approach recognizes that the production of atmospheric secondary particles from non-NPF events (days with no apparent particle growth) is becoming more significant in a world with growing anthropogenic influence (Kulmala et al., 2022a). Such a measure would increase the applicability of our models on a global scale.
The observed particle formation rates (J5) at 5 nm were calculated from the measured PNSD according to Eq. (1) (Kulmala et al., 2012).
The first term is the change in concentration in the size bin, 5–9 nm. Ideally, this term and the concentration of particles within the size range, , in the following terms are associated with the growth of particles formed by atmospheric NPF past 5 nm; however, especially in traffic-related environments, they may also have an unknown contribution by direct particle emissions to this respective size range (Okuljar et al., 2021; Rönkkö et al., 2017). The second term is the coagulation sink, which describes the 5–9 nm particle losses due to coagulation with larger particles calculated from the PNSD at each measurement site (Kulmala et al., 2012). The third term describes the loss of particles due to their growth out of the size bin. Here, we calculated the growth rates (GRs) of 5–9 nm particles using the maximum concentration method (Kulmala et al., 2012) for days classified as NPF event days as described by Dal Maso et al. (2005). The GR for non-event days was approximated using the normalized PNSD from the sum of non-NPF events at each site. Such an approximation is validated for several locations as a “quiet NPF” occurs with the similar GR to that on NPF event days (Kulmala et al., 2022a).
2.3.2 Extrapolation of particle formation rates
For Budapest and Manacapuru (Amazonian basin), the particle formation rates were calculated from PNSD measurements at 6 and 10 nm, respectively. Therefore, we obtained J5 by extrapolating from J6 and J10, respectively. The J5 extrapolation followed the analytical formula derived by Kerminen and Kulmala (2002). We extrapolated J5 from J6 for Budapest. For Manacapuru, the extrapolations were done separately for J10 (wet season) and J14 (dry season), due to the particle size limit of the measurement instrument.
2.3.3 Condensation and coagulation sink (CS and CoagS)
The CS and CoagS were calculated from the measured PNSD data for each site using the method proposed by Kulmala et al. (2012). To ensure the comparability between all of the locations, both CS and CoagS were calculated without the correction for hygroscopic growth. There are several ways to determine the hygroscopic growth factors in CS and CoagS calculations. Laakso et al. (2004) developed parameterizations for Hyytiälä based solely on the meteorological conditions and aerosol composition in Hyytiälä, which results in the inapplicability of that method to other sites. In the Supplement of Baalbaki et al. (2021), Fig. S4 shows that the CS with hygroscopic correction is about 1.1–1.3 times higher than the dry CS, which would result in an overestimation of CS for the case of Cyprus. Petters and Kreidenweis (2007) introduced the single hygroscopicity parameter κ (kappa), which can be derived from a humidified tandem differential mobility analyzer (HTDMA) or cloud condensation nuclei counter measurements, or it can be based on aerosol chemical composition obtained from instruments such as the Aerosol Chemical Speciation Monitor (ACSM) or aerosol mass spectrometers (AMSs). In other locations, since organics are typically the dominant component of aerosol mass in continental areas or marine polluted areas (Chen et al., 2022) and are less hygroscopic than inorganics, one can expect an underestimation of CS similar to the one reported in Baalbaki et al. (2021). As a result, we omitted the hygroscopic growth impact for the chosen measurement sites to harmonize the data composition and the later model analysis.
2.3.4 Training and testing datasets
The parameterizations were developed using the combined dataset from all six measurement sites at an hourly time resolution. Data points were selected by considering the detection limits of the instruments, and, therefore, the filters were set to J5 > cm−3 s−1, H2SO4 concentration > 5×103 cm−3, RH ∈ [0,100] %, and CS > s−1. The complete dataset was then randomly resampled into a training set (75 % of the complete dataset) and a testing set (25 % of the rest of the complete dataset) for parameterization. In model testing, we included two additional inputs from H2SO4 concentration proxies developed by Dada et al. (2020) for Hyytiälä and Beijing. The detailed numbers of data points per site are shown in Table 1. The data distribution and comparison of each input variable are displayed in Fig. S2 in the Supplement, where the overall variations of the input variables across the six sites are distinct in their range and intensity, which emphasizes the inclusivity of model training for wider application in global environments.
3.1 Derivation of parameterization models
We derived the parameterized J5 based on the input variables (H2SO4, RH, and CS), which were chosen based on field observations that highlighted their roles in the particle formation mechanism across various environments (Baalbaki et al., 2021; Dada et al., 2020; Kerminen et al., 2018; Myers et al., 2022; Salma et al., 2016, 2021; Yan et al., 2021). It has been discovered that NPF events occur favorably under lower RH, e.g., in boreal forests (Dada et al., 2018; Yao et al., 2018), Mediterranean regions (Debevec et al., 2018), the CLOUD chamber experiment (Duplissy et al., 2016), and model studies (Hamed et al., 2011). RH was shown to be seasonally related to cloudiness and global radiation, so that decreasing global radiation can lead to increased RH and cloudiness within the troposphere (Ruosteenoja and Räisänen, 2013). To reduce the model complexity, we opted to use RH as an indirect indicator of global radiation. A lower CS facilitates the occurrence of NPF events, even in contrasting environments with distinct types of condensable vapor. For example, CS is a measure of a sink for anthropogenic vapors in a megacity (Wang et al., 2011), biogenic vapors in a clean boreal forest (Dada et al., 2017; Tuovinen et al., 2020), and growing sub-5 nm clusters and particles (Kulmala et al., 2017). When combined with H2SO4 as an input variable, the evidently important sink effect of a pre-existing particle population on the ambient H2SO4 concentration is implicitly transferred from CS to H2SO4 in our parameterization. Indirectly, CS may also be associated, either causally or not, with (1) emissions or sinks of vapors other than H2SO4 participation in NPF or particle growth and (2) primary particle emissions from traffic, which would influence particle formation rates estimated from observations using Eq. (1). Furthermore, since we omit the influence of hygroscopic growth of particles on CS, a fraction of the real sink effect of CS is implicitly transformed into the variable RH in our parameterization.
We tested with T as an input variable during model derivation and training. However, the modeled results did not show improvement compared to the current parameterization, suggesting that T provided redundant information for describing particle formation in the context of our model's global application.
Other than sulfuric acid, highly oxygenated organic molecules (HOMs) and ammonia (NH3) have been found to play a significant role in particle formation processes (Bianchi et al., 2019; Lehtipalo et al., 2018). The possible cluster types may include H2SO4–NH3–H2O (Yu et al., 2018) and HNO3-related clusters such as HNO3–H2SO4–NH3 in the upper-tropospheric particle nucleation (Wang et al., 2020, 2022, 2023). However, we are unable to include HOM or NH3 concentrations owing to limited data availability from the chosen measurement sites. So far, long-term measurements (> 1 year) of HOMs, matching the time range covered by other variables, are only available in Hyytiälä from a CI-APi-ToF mass spectrometer. However, this is not the case at the other sites, limiting our ability to have simultaneous HOM data across all of the environments included in this study. Similarly, the NH3 concentrations either did not cover the same time period as the other variables or were unavailable for the other environments.
Different versions of the parameterization models
The derived model functional forms are as follows.
Model 1 (the baseline model, Eq. 2) presents the simplest particle formation mechanism based solely on the abundance of the precursor vapor H2SO4 concentrations in the atmosphere. The coefficient k1 serves as a scaling coefficient that represents the activation rate of clusters in the presence of H2SO4 molecules during cluster formation (Kulmala et al., 2006; Paasonen et al., 2010).
Model 2 (Eq. 3) introduces RH in addition to model 1 to partially represent the effect of the changing meteorological conditions relating to the global radiation and ambient water vapor content on J5 in general in different types of environments (Dada et al., 2017; Hamed et al., 2011; Li et al., 2019). The coefficient k2 serves as a scaling coefficient and is shown as the activation efficiency of the nucleated clusters.
Model 3 (Eq. 4) includes, in addition to model 2, the factor CS. As discussed above, in our parameterization CS is connected not only to the sink of newly formed particles prior to their growth past 5 nm, but possibly also to sinks or sources of vapors other than H2SO4 participating in particle formation and growth and, in polluted environments, sub-10 nm particle emissions from traffic. The coefficient k3 serves as a scaling coefficient for the activation and survival efficiency of the nucleated clusters.
Model 4 (Eq. 5) additionally accounts for the formation of H2SO4 multimers in the gas phase prior to cluster formation as assumed by the kinetic theory (McMurry and Friedlander, 1979). The coefficient kSA represents the number of H2SO4 molecules (2, 3, 4, etc.). Therefore, k4 in this case is not the activation coefficient anymore but includes both the collision frequency and the probability of a stable particle formation after the collision (Sihto et al., 2006; Weber et al., 1996).
3.2 Model training results
To derive a parameterized J5 based on precursor and other input variables from the training dataset, we used the fmincon optimization algorithm in MATLAB to retrieve the values of each coefficient (k1–k4, kSA, kRH, and kCS) from the training dataset. The coefficients obtained for each of the models can be found in Table 2. The derived models with the optimized coefficients were applied to the testing datasets and compared with the observed J5 and the parameterized J5. We evaluated the performance of each model based on the data distribution, the resulting deviation from observations, and the model uncertainty. To maintain the global model's simplicity, the parameterization covered both daytime and nighttime data for all of the sites in all of the models.
Table 2Coefficient values (kx, kRH, kCS, and kSA) retrieved from parameterizations using the training dataset. The term “SSe” represents the sum of the squared error of each model. The units of kx (x = 1, 2, 3, and 4) vary as the functional form of the model changes, while kRH, kCS, and kSA do not contain units. Since RH is counted using percentage (%), a dimensionless number, the scaling coefficients k0 mainly count the units from H2SO4 concentrations and CS. As such, we must ensure that the RH input is in its percentage.

Figure S3 presents the measured to modeled J5 from models 1 to 4 using training datasets from six measurement sites, including the slopes and coefficient of determination (R2). Overall, by comparing model 1 (Fig. S3a) and model 2 (Fig. S3b), we observed an improvement in the model performance with the inclusion of RH. The R2 value improved from 0.28 to 0.44, and the slope increased from 0.29 to 0.56. This observation confirmed the importance of considering meteorological impact when parameterizing J5. By including CS in model 3, the model improved further (Fig. S3c), with R2 increasing from 0.44 to 0.49 and the slope from 0.56 to 0.62. To further introduce the kinetic theory and the formation of H2SO4 dimers and other multimers, we added an exponent over H2SO4 in model 4 (Fig. S3d). This addition showed a further improved correlation and slope between the measured or modeled data for the training datasets (R2 = 0.57, slope = 0.76). In subsequent testing, model 4 generally outperformed the other models (see Sect. 4 and Fig. 2).
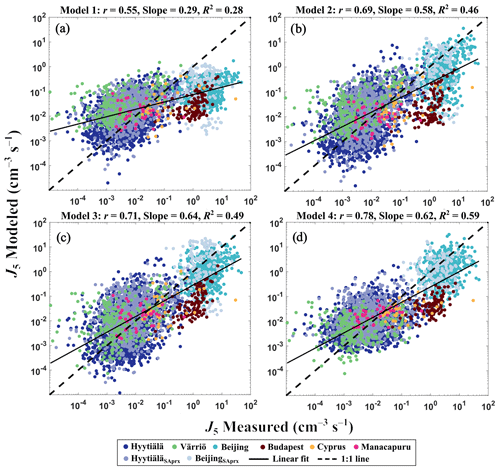
Figure 2Modeled and measured J5 scatterplots in log-scale from four models using the testing dataset containing data from all six sites at hourly time resolution. Each color represents the data from one measurement site, including datasets with H2SO4 proxy data from Hyytiälä and Beijing. The straight line shows the robust linear fit between the modeled and measured J5 values in log-scale, and the dashed line represents the 1 : 1 line. The correlation coefficient r, the slope of the linear fit, and the coefficient of determination R2 are shown in the title of each subplot.
3.3 Model evaluations
3.3.1 Mean absolute error (MAE) and root mean square error (RMSE)
We computed the MAE and RMSE for each model using the testing dataset to gain a better understanding of the models' performance. The numerical values of the MAEs and RMSEs are given in Table S3.
The MAE calculation equation is as follows:
where n is the number of data points (here it is the total number of data points from the testing set; see Table 1), yi is the observed value, and denotes the predicted value. The MAE measures the accuracy of the models' prediction power by quantifying the average magnitude of errors between observed and predicted values (Chai and Draxler, 2014). A lower model error is manifested by a lower MAE value.
The RMSE is calculated as the square root of the difference between the measured (yi) and predicted () J5 values normalized by the number of data points.
The RMSE also measures the average magnitude of the errors of models. However, unlike the MAE, the RMSE squares the errors, giving greater weight to larger errors and penalizing them more heavily (Chai and Draxler, 2014). Therefore, RMSE values reveal whether the models' performances are highly influenced by large prediction errors. Similar to the MAE, lower RMSE values indicate better model performance.
Figure S4 (upper panel) depicts a declining trend of the overall MAE from models 1 to 4 (Eqs. 2–5). For the environmental types investigated in this study, the MAE values of the four models from all of the sites are lower than 1, indicating that the mean differences in magnitude for J5 are minor when utilizing the parameter settings from our models. However, Budapest stands out due to the apparently higher MAE, potentially highlighting the distinct NPF mechanism in Budapest compared to the other sites as well as the seasonal limitations in its data (spring 2018 only).
The RMSE values increased as more parameters were added to the model, peaking for model 3 (Fig. S4, lower panel), even though model 3 can predict J5 for multiple types of environments at a satisfactory level. We can see that, from model 1 to model 2, the inclusion of RH increased the model errors more compared to the addition of CS from model 2 to model 3. However, the RMSE values dropped significantly when H2SO4 was allowed to vary with an exponent kSA in model 4 in the presence of both RH and CS.
Based on the results summarized above, models 3 and 4 (Eqs. 4 and 5) seem to be the most promising for global J5 prediction among all the model types owing to their low MAE values. However, the lower RMSE for model 4 showed better performance compared to model 3.
3.3.2 Akaike information criterion
The Akaike information criterion (AIC) is a statistical measure that helps to evaluate the goodness of fit of a statistical model. We use the AIC as an evaluation tool because it can evaluate models with different numbers of parameters and complexities, ensuring a balanced assessment. Eventually, it allows us to select the model with the best balance between the model complexity and goodness of fit. The parameters used to calculate the AIC for each site are shown in Tables S4–S6. A lower AIC score indicates a superior goodness of fit and a lower tendency for model overfitting. The relative likelihood term (L, L = ), calculated from AIC scores, reflects the likelihood that the ith model will minimize information loss as compared to the model with the lowest AIC. A relative likelihood of 1 suggests that the model exceeds other models in minimizing information loss. For boreal forest environments (Table S4) and urban environments (Table S5), models 1 and 4 both minimized information loss the most. For rural regions, model 4 (Eq. 5) performs the best (Table S6). Compared to the baseline model (model 1, Eq. 2), we find that H2SO4 is a more powerful parameter than RH or CS in all environments. However, in Manacapuru, including RH and CS clearly shows an improved predictive accuracy in model 4 (Eq. 5).
4.1 Parameterization testing results
The scatterplots (Fig. 2) demonstrate the overall performance of the parameterizations from the four models (Eqs. 2–5) using the testing dataset. The overall and site-specific Pearson coefficients, slopes from robust linear fits between the measured and modeled J5, and the number of data points from the testing dataset can be found in Table 3. Overall, r increased significantly for models 2 and 3 (Fig. 2b and c, r = 0.69, r = 0.71) compared to model 1 (Fig. 2a, r = 0.55) as we include relative humidity and condensation sink as model parameters. Model 4 provides the best linear fit results, implying that the model can predict an overall reliable estimation of J5 in all the investigated environment types (Fig. 2d, r = 0.78). It is notable that, with the combined datasets, the condensation sink receives a positive exponent in models 3 and 4 (kCS = 0.56 and 0.67, respectively), likely due to its association with concentrations of condensable vapors other than H2SO4 and traffic emissions.
Table 3Summary of the overall and site-specific correlation coefficients (r) of the four models using the testing dataset. The numbers in parentheses under the site names represent the count for the data points.
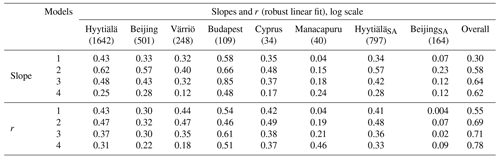
4.1.1 Boreal forests: Hyytiälä and Värriö
Given the boreal forest background, Hyytiälä and Värriö exhibited comparable variations in the distribution of modeled J5 values from the four model types. As shown in Fig. 3, model 3 (a3, b3, c3) and model 4 (a4, b4, c4) illustrated a more centered data distribution between the modeled and measured J5, which demonstrates a potential favoring of NPF under low-RH conditions likely associated with increased global radiation for the boreal forest environment (Dada et al., 2018; Hamed et al., 2011) and lower sinks for vapors and growing sub-5 nm particles (see Sect 3.1). Note that the mean H2SO4 concentration in Värriö is about twice as high as that in Hyytiälä, in contrast to CS, which is clearly lower in Värriö (Table S2). The low CS in Värriö compared with Hyytiälä is primarily due to the lower emission rate of the regional precursor vapors (e.g., Tunved et al., 2006), also leading to the lower observed NPF event frequencies (Kyrö et al., 2014; Neefjes et al., 2022). We must note that the Hyytiälä data spanned 3 years, containing more data points for model training, whereas the Värriö data only covered the period from April to August 2019, excluding the entire cold season, when H2SO4 concentrations are significantly lower than those during the warm season (Jokinen et al., 2022). As a result, our models 3 (Fig. 3a3, b3, c3) and 4 (Fig. 3a4, b4, c4) can predict J5 for boreal forest environments at a satisfactory level, including the possibility of using the estimated H2SO4 concentration from proxies as input. Nevertheless, limitations regarding the precursor vapor production rate could potentially influence the prediction accuracy.
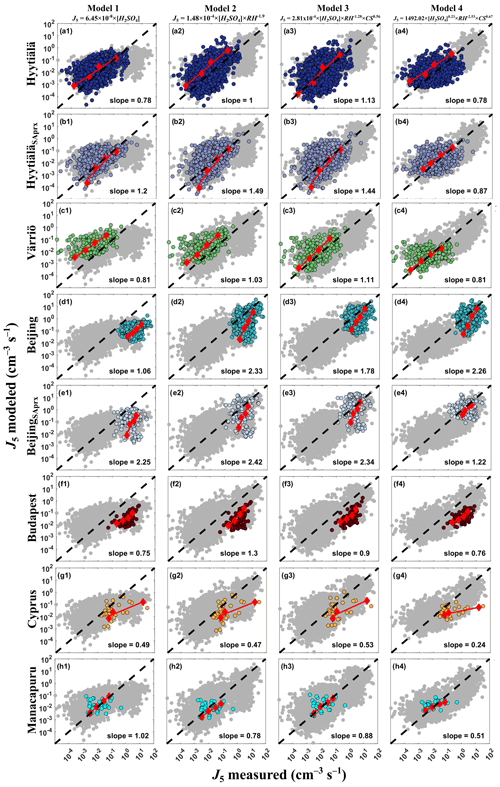
Figure 3Modeled and measured J5 scatterplots in log-scale from four models using the testing dataset containing data from all sites at hourly time resolution. The labels on the left side of the y axis are the site names. The subscripted label “SAprx” indicates that the input H2SO4 concentrations were from H2SO4 proxies. The light-grey scatters are all data points from the testing dataset, and the colored scatters on top of them indicate the results from the corresponding measurement site. The red diamonds are the binned daily medians to show the temporal aggregation of the model performances on daily data. Overall, a daily scale presents excellent performances on model 4 for boreal forest environments (a4, b4, c4), polluted cities (d4, e4), and an organic-vapor-dominated high-humidity region (h1–h3). The red solid lines represent the linear fit on the binned hourly medians. The dashed line is the 1:1 line.
4.1.2 Urban-influenced: Beijing and Budapest
In an anthropogenic-emission-dominated region such as Beijing, the measured and modeled J5 are well-aligned around the 1:1 line using model 3 (Fig. 3d3, e3, f3) and model 4 (Fig. 3d4, e4, f4). In Beijing, a polluted megacity, the dominating precursor type has been found to be H2SO4–amine clusters (Cai et al., 2021). As expected, the testing result showed dramatic underestimations for Beijing using model 1, with only H2SO4 concentrations considered (Fig. 3d1), whereas models 2 (Fig. 3d2) and 3 (Fig. 3d3) yielded clearly enhanced J5 predictions, with relatively minor differences between models 2 and 3. These features are consistent with the fact that, in addition to H2SO4, other vapors are also important to NPF and sub-5 nm particle growth in Beijing and demonstrate that RH and CS together in our parameterization determine in a complicated way the sources and sinks of these vapors, the survival probability of sub-5 nm particles, and the potential emissions of sub-10 nm primary particles from traffic. This study did not include amine-related compounds in the formulas because the lack of measured NH3 data makes parallel comparisons difficult between the sites chosen for model training.
For Budapest, a large European city, the underestimates in the modeled J5 are not as much improved as they were for Beijing when including RH or CS in the parameterization, which is indicative of distinct particle formation pathways between Beijing and Budapest, even though both sites represent urban background environments. On the one hand, it is worth noting that including RH (model 2, Eq. 3) resulted in a decrease in the correlation coefficients between the measured and modeled J5 in Budapest from 0.54 to 0.46 (Table 3). This suggests that the role of RH in the NPF process in Budapest is less significant than the other chosen inputs, despite previous indications that high RH levels have a strong potential to suppress NPF during non-event days in Budapest (Salma et al., 2021), even though the RH values in Budapest were considerably higher than those in Beijing (Table S2). On the other hand, including CS (model 3, Eq. 4) in addition to RH (model 2, Eq. 3) leads to an increase in the correlation coefficients between the measured and modeled J5 from 0.46 to 0.61 (Table 3). We used both NPF and non-NPF days during model training even though it was found that CS was about 50 % higher during non-NPF events in Budapest than the values during NPF events (Salma et al., 2016). As a result, it is difficult to determine whether the model's performance gain was entirely brought on by the addition of CS. Otherwise, the results are all in line with the earlier indirect evidence that chemical species other than H2SO4 influence the particle growth and possibly NPF process in Budapest (Salma and Németh, 2019). If one considers additional vapors other than H2SO4 for Budapest alone for J parameterization, one could include oxidation products of VOCs originating from either urban vegetation emissions or traffic emissions. For example, isoprene oxidation products can be used to describe the inhibiting effect on NPF (Heinritzi et al., 2020; Kiendler-Scharr et al., 2009), while monoterpene oxidation products could enhance sub-3 nm particle growth (Kulmala et al., 2013).
Based on the testing results, model 3 is more likely to predict a more accurate J5 for Beijing based on the highest AIC ratio (except for model 1), while model 1 predicts better for Budapest. Note the fact that J5 showed distinct levels of measured J5 dependence with RH and CS in Beijing and Budapest (J5 and RH – Beijing: r = −0.21, Budapest: r = −0.1; J5 and CS – Beijing: r = −0.02, Budapest: r = 0.57; Fig. S1).
4.1.3 Mediterranean rural site: Agia Marina, Cyprus
For Cyprus, it appears that meteorology and condensation sink terms have only minor effects on the formation of 5 nm particles in such a rural environment under the influence of marine vapors when comparing the results from the testing dataset across the models (Fig. 3g). However, including RH slightly improves the correlation between the modeled and measured J5 as seen in model 2 (r increases from 0.42 to 0.49, Table 3). The reduced values of r in model 3 (Eq. 4) indicate a somewhat reversed impact of CS on J5, which requires additional examination as J5 and CS are weakly correlated (r = 0.03, Fig. S1). The H2SO4 concentration showed a low contribution to J5, with the exponent being less than 1 in model 4 (Table 3), which led to a more underestimated modeled J5 compared to model 1 (Fig. 3g1, g4). This could be an indication that potentially other anthropogenic, biogenic, or marine compounds make greater contributions to the particle formation processes in Cyprus than H2SO4 (Debevec et al., 2018). Owing to the orographic conditions, the air mass types approaching the Cyprus measurement site are mixed, including the ones from northern Africa, marine environments, Europe, and northwestern and southwestern Asia. This results in the Mediterranean atmosphere in Agia Marina containing various vapors that could influence NPF. The potential key contributors could include oxidation products of dimethyl sulfide (DMS) originating from ocean plankton emissions (Rosati et al., 2021), iodine oxidation products like HIO3 (He et al., 2021), the stabilizing agent NH3 (Jiang and Xia, 2017; Lan et al., 2021; Lehtipalo et al., 2018; Yu et al., 2018), and oxidation products of VOCs from the surrounding pine forests and oaks under favorable meteorological conditions (Debevec et al., 2018).
We should note that the measurements in Cyprus covered only 2 weeks in springtime, which limited our quantitative observations in model training for other seasons compared to sites with long-term measurements. Based on the findings above, model 1 seems to be the most suitable functional form for the prediction of J5 in Cyprus.
4.1.4 Amazonian basin: Manacapuru, Brazil
The measured and modeled values of J5 from the Manacapuru site scatter around the 1:1 line in all the models (Fig. 3). Previous studies reported high RH levels year-round at the measurement site near Manacapuru (Myers et al., 2022; Zhao et al., 2022), which is expected to suppress NPF frequency and lead to lower formation rates. We observed such a suppression effect when taking RH into account, as shown by the increased correlation coefficients from 0.04 to 0.19 (Table 3). Studies from Manacapuru suggested that the epoxide vapors could be a potential precursor vapor in particle formation because of anthropogenic influences (Paulot et al., 2009), while Xu et al. (2014) suggested that the presence of epoxide vapors can enhance particle nucleation when RH levels increase. We did not observe an apparent improved model performance in model 3 when CS is included, as r remained almost unchanged compared to model 2 (Table 3). One factor to consider is that we did not apply the hygroscopic growth factor when calculating CS for Manacapuru to maintain the consistency of the training dataset. However, the impact of RH on CS, particularly on the actual particle surface area available for H2SO4 uptake, seems to be significant for high-RH environments like Manacapuru (Myers et al., 2022). Another assumption could be that, even with the high CS, it is still low enough to allow sufficient precursor vapors contributing to NPF processes.
These current findings provide evidence for H2SO4 being an effective enough precursor for the particle formation at 5 nm in the atmosphere of Manacapuru (model 1, Fig. 3h1). However, the RH stabilization effect on H2SO4 is not necessarily exerted, as RH remains at high values of around 89 ± 13 % regardless of whether it is measured during the wet season or the dry season (Myers et al., 2022). With these observations, model 1 with a focus on the H2SO4 concentrations manages to predict J5 well for a biogenic-vapor-dominated environment like Manacapuru.
4.2 Tracer Model 5 simulation
We simulated the PNSD in the EC-Earth global chemistry transport model TM5-MP (Tracer Model 5, massively parallel version; details can be found in the Supplement) by applying it with our J5 models 1 and 4. Together, we compared our simulation results with the acid–organic vapor binary homogeneous nucleation model of Riccobono et al. (2014):
where km = cm6 s−1, p = 2, and q = 1.
The details of the 14 tested measurement stations are shown in Table S7. Note that the data from these 14 stations are independent of any training or testing datasets used in the previous sections of this paper. Here, we essentially compared the simulated and measured PNSDs in three particle modes (nucleation, Aitken, and accumulation) from the entire year of 2018 to assess the simulation accuracy among global environments.
Figure 4 shows the comparisons of PNSDs between the on-site measurements and the TM5-MP simulations. For biogenic environments, simulations using model 4 show the particle number size distribution closest to the measured ones, particularly in Aitken-mode particles, promoting the sulfuric-acid-based nucleation mechanism involving the source–sink meteorology, even for environments dominated by biogenic vapors. For the Arctic region, particle concentrations simulated by model 4 are overestimated overall, while model 1 simulation shows better alignment of particle number concentrations around the Aitken mode. This might indicate that the nucleation process has a lower dependence on the variations of meteorology than we expected. For coastal environments, even though Utö (Baltic Sea island) and La Réunion (Southern Hemisphere island) are located in different hemispheres and have different geographical settings, the nucleation mechanisms from models 1 and 4 both show similar predictions of particle concentrations across particle modes, with larger underestimation in the accumulation mode for model 1. This once again validates the source–sink meteorology mechanism in model 4. By observing the ratio between the simulated and measured particle number concentrations, we can quickly see that the sulfuric-acid-based particle formation mechanisms with (model 4) or without (model 1) meteorology inputs have successfully narrowed the gap between the simulations and observations across all of the particle modes, with significant improvements for the nucleation mode (Fig. 5). The “Total” contains the simulated / measured particle number concentration ratio from all of the particle modes, and it is clearly seen that applying model 4 improves the overall global PNSD simulation compared with the sulfuric acid–organic vapor binary model of Riccobono et al. (2014). This observation shows that including the RH and CS is needed for better understanding of the global particle number size distributions.
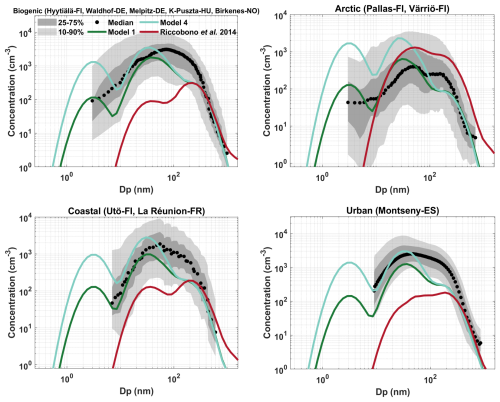
Figure 4Environment-specific TM5-MP-simulated particle number size distribution from 2018 in annual medians. Biogenic sites include rural and regional background environments (Hyytiälä, Waldhof, Melpitz, K-Puszta, and Birkenes). Coastal sites cover islands in the Baltic Sea and in the Indian Ocean in the Southern Hemisphere close to Madagascar (Utö and La Réunion). The Arctic sites are two Finnish sites both situated within the Arctic Circle (Pallas and Värriö). The urban site is represented by the Spanish city of Montseny.
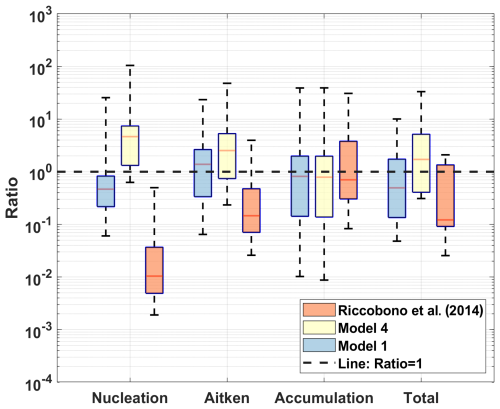
Figure 5Ratio of simulated and measured particle number concentrations from the 14 global sites from TM5-MP simulations in three nucleation settings (Riccobono, model 1, and model 4), resulting in three particle modes (nucleation, Aitken, and accumulation) in 2018 annual medians. The black line represents “ratio = 1” as a reference line. The “Total” represents the overall ratio between the simulation and the measurement particle number concentrations from all of the modes.
The particle formation rate is one of the key characteristics in new particle formation studies. By utilizing distinct field measurement data, we can model the particle formation rate and estimate the overall atmospheric aerosol budget over different environments. We parameterized J5 in four functional forms using the combined datasets from six environments, covering boreal forests (Hyytiälä and Värriö), urban sites (the megacity of Beijing and the large European city of Budapest), and rural environments (Cyprus and Manacapuru). The particle formation schemes involve the main precursor vapor H2SO4, relative humidity (RH), and a condensation sink (CS). Due to the small number of parameters and the diversity of environments included to generate the schemes, the roles of RH and CS are related not only to their potential direct impact on J5, but also sources and sinks of vapors other than H2SO4 contributing to the formation and growth of sub-5 nm particles and potential emissions of sub-10 nm particles, e.g., from traffic. Overall, our models showed improved performances as RH and CS were taken into consideration. The model evaluations may suggest that the particle formation mechanism is more sensitive to certain factors in specific environments. Sulfuric acid is an effective precursor vapor in NPF processes for most of the measurement sites we selected for model training. Nevertheless, relying solely on H2SO4 generally resulted in a weaker model performance for environments where the NPF schemes are dominated by biogenic emissions. This suggests that, to develop globally applicable particle formation rate models, more precursor vapor types need to be included alongside H2SO4.
The purpose of the paper is twofold: first, to address the lack of knowledge regarding global particle formation rates for particles at 5 nm and larger, and second, to provide a globally applicable semi-empirical parameterization for the sulfuric-acid-based neutral particle formation. The simplicity of the parameterization is demonstrated by three factors. First, NPF is a widespread occurrence in various types of environments, where the characteristics of particle formation share common mechanisms involving major precursor types and environmental factors. Second, the main input H2SO4 concentration data can be obtained from field measurements or proxies, from which the contribution of H2SO4 to NPF can be directly compared among global sites. Third, we skip the microphysics complexity of sub-5 nm particles, where the physical and chemical properties differ significantly from those that are above 5 nm when discussing particle formation and growth.
The limited data availability from certain sites (less than 1 year), such as Budapest, Cyprus, and Manacapuru, should be noted when applying our models. Conclusions drawn from these sites can be more confidently applied to the specific seasons covered in the model training, such as spring being more representative of Budapest and Cyprus and summer to early fall being more representative of Manacapuru.
Overall, our parameterization findings show that our models including H2SO4 concentration, RH, and CS can predict J5 at a satisfactory level for various environment types at once. Among the tested models, models 3 and 4 (Eqs. 4 and 5) can be utilized to predict J5 on a global scale if (1) the H2SO4 concentrations are known, whether through field measurement or proxies; (2) the meteorology parameter RH is monitored continuously; and (3) the particle number size distributions are sufficient and assessed to yield CS. Some caution should be maintained when utilizing these models for environments with very low RH and/or high CS, especially if the high CS is related to primary particle emissions, as the associations between these model parameters and J5 are complicated and multifaceted. While the parameterizations presented in this study offer an improvement over previous approaches, further development is needed to incorporate vapors important for NPF, such as iodine oxo-acids, particularly in marine environments.
The MATLAB code used for the parameterization training in this paper is available on Zenodo at https://zenodo.org/records/15295592 (Li, 2025).
The datasets used in this study are now available on Zenodo at https://zenodo.org/records/15295592 (Li, 2025). The data are licensed under a Creative Commons 4.0 Attribution (CC BY) license. The data for the 14 global measurement sites are from the EBAS database (https://ebas-data.nilu.no/, NILU, 2024).
The supplement related to this article is available online at https://doi.org/10.5194/ar-3-271-2025-supplement.
Measurements: NS, RB, CY, LQ, TJ, IS, MV, TW. Data analysis: XL, LD, MZ, NS, RB, CY, LQ, IS, PZ. Result interpretation: XL, LD, PP, TN. Discussions: all the co-authors. Writing: XL, LD, PP, VMK, TN. Comments and revisions: all the co-authors.
At least one of the (co-)authors is a member of the editorial board of Aerosol Research. The peer-review process was guided by an independent editor, and the authors also have no other competing interests to declare.
Publisher’s note: Copernicus Publications remains neutral with regard to jurisdictional claims made in the text, published maps, institutional affiliations, or any other geographical representation in this paper. While Copernicus Publications makes every effort to include appropriate place names, the final responsibility lies with the authors.
This work was supported by European Research Council H2020 projects GASPARCON (grant no. 714621), FORCeS (grant no. 821205), and EMME-CARE (grant no. 856612); by the Cyprus Government; by the Hungarian Research Development and Innovation Office (contract Advanced 150835); by the Research Council of Finland with grant nos. 355330 and 360114; and by ACCC Flagship funded by the Research Council of Finland (grant no. 337549). Lubna Dada received funding from the Swiss National Science Foundation (Ambizione grant no. 216181). We are grateful to all the people who contributed to the ambient measurements at the measurement stations. The Finnish and Cypriot stations are part of the European research infrastructure ACTRIS. We acknowledge the ACTRIS CiGas (Centre for Reactive Trace Gases In Situ Measurements) and CAIS-ECAC (Centre for Aerosol In Situ Measurements) for providing operational support for the aerosol and reactive trace gas instruments at the stations. We also thank James N. Smith for providing the GoAmazon2014/5 data for Manacapuru.
This research has been supported by the European Research Council, EU Horizon 2020 research program (grant nos. 714621, 856612, and 821205); the Research Council of Finland (grant nos. 355330 and 360114); the Schweizerischer Nationalfonds zur Förderung der Wissenschaftlichen Forschung (grant no. 216181); the National Research, Development and Innovation Office (grant no. 150835); and the ACCC Flagship funded by the Research Council of Finland (grant no. 337549).
This paper was edited by Luis A. Ladino and reviewed by two anonymous referees.
Aalto, P., Hämeri, K., Becker, E., Weber, R., Salm, J., Mäkelä, J. M., Hoell, C., O'dowd, C. D., Hansson, H.-C., Väkevä, M., Koponen, I. K., Buzorius, G., and Kulmala, M.: Physical characterization of aerosol particles during nucleation events, Tellus B, 53, 344–358, https://doi.org/10.3402/tellusb.v53i4.17127, 2001.
Baalbaki, R., Pikridas, M., Jokinen, T., Laurila, T., Dada, L., Bezantakos, S., Ahonen, L., Neitola, K., Maisser, A., Bimenyimana, E., Christodoulou, A., Unga, F., Savvides, C., Lehtipalo, K., Kangasluoma, J., Biskos, G., Petäjä, T., Kerminen, V.-M., Sciare, J., and Kulmala, M.: Towards understanding the characteristics of new particle formation in the Eastern Mediterranean, Atmos. Chem. Phys., 21, 9223–9251, https://doi.org/10.5194/acp-21-9223-2021, 2021.
Bellouin, N., Quaas, J., Gryspeerdt, E., Kinne, S., Stier, P., Watson-Parris, D., Boucher, O., Carslaw, K. S., Christensen, M., Daniau, A.-L., Dufresne, J.-L., Feingold, G., Fiedler, S., Forster, P., Gettelman, A., Haywood, J. M., Lohmann, U., Malavelle, F., Mauritsen, T., McCoy, D. T., Myhre, G., Mülmenstädt, J., Neubauer, D., Possner, A., Rugenstein, M., Sato, Y., Schulz, M., Schwartz, S. E., Sourdeval, O., Storelvmo, T., Toll, V., Winker, D., and Stevens, B.: Bounding Global Aerosol Radiative Forcing of Climate Change, Rev. Geophys., 58, e2019RG000660, https://doi.org/10.1029/2019RG000660, 2020.
Bergman, T., Makkonen, R., Schrödner, R., Swietlicki, E., Phillips, V. T. J., Le Sager, P., and van Noije, T.: Description and evaluation of a secondary organic aerosol and new particle formation scheme within TM5-MP v1.2, Geosci. Model Dev., 15, 683–713, https://doi.org/10.5194/gmd-15-683-2022, 2022.
Bianchi, F., Kurtén, T., Riva, M., Mohr, C., Rissanen, M. P., Roldin, P., Berndt, T., Crounse, J. D., Wennberg, P. O., Mentel, T. F., Wildt, J., Junninen, H., Jokinen, T., Kulmala, M., Worsnop, D. R., Thornton, J. A., Donahue, N., Kjaergaard, H. G., and Ehn, M.: Highly Oxygenated Organic Molecules (HOM) from Gas-Phase Autoxidation Involving Peroxy Radicals: A Key Contributor to Atmospheric Aerosol, Chem. Rev., 119, 3472–3509, https://doi.org/10.1021/acs.chemrev.8b00395, 2019.
Bousiotis, D., Pope, F. D., Beddows, D. C. S., Dall'Osto, M., Massling, A., Nøjgaard, J. K., Nordstrøm, C., Niemi, J. V., Portin, H., Petäjä, T., Perez, N., Alastuey, A., Querol, X., Kouvarakis, G., Mihalopoulos, N., Vratolis, S., Eleftheriadis, K., Wiedensohler, A., Weinhold, K., Merkel, M., Tuch, T., and Harrison, R. M.: A phenomenology of new particle formation (NPF) at 13 European sites, Atmos. Chem. Phys., 21, 11905–11925, https://doi.org/10.5194/acp-21-11905-2021, 2021.
Brean, J., Beddows, D. C. S., Harrison, R. M., Song, C., Tunved, P., Ström, J., Krejci, R., Freud, E., Massling, A., Skov, H., Asmi, E., Lupi, A., and Dall'Osto, M.: Collective geographical ecoregions and precursor sources driving Arctic new particle formation, Atmos. Chem. Phys., 23, 2183–2198, https://doi.org/10.5194/acp-23-2183-2023, 2023.
Cai, R., Yan, C., Yang, D., Yin, R., Lu, Y., Deng, C., Fu, Y., Ruan, J., Li, X., Kontkanen, J., Zhang, Q., Kangasluoma, J., Ma, Y., Hao, J., Worsnop, D. R., Bianchi, F., Paasonen, P., Kerminen, V.-M., Liu, Y., Wang, L., Zheng, J., Kulmala, M., and Jiang, J.: Sulfuric acid–amine nucleation in urban Beijing, Atmos. Chem. Phys., 21, 2457–2468, https://doi.org/10.5194/acp-21-2457-2021, 2021.
Cai, R., Deng, C., Stolzenburg, D., Li, C., Guo, J., Kerminen, V.-M., Jiang, J., Kulmala, M., and Kangasluoma, J.: Survival probability of new atmospheric particles: closure between theory and measurements from 1.4 to 100 nm, Atmos. Chem. Phys., 22, 14571–14587, https://doi.org/10.5194/acp-22-14571-2022, 2022.
Calvo, A. I., Alves, C., Castro, A., Pont, V., Vicente, A. M., and Fraile, R.: Research on aerosol sources and chemical composition: Past, current and emerging issues, Atmos., 120–121, 1–28, https://doi.org/10.1016/j.atmosres.2012.09.021, 2013.
Chai, T. and Draxler, R. R.: Root mean square error (RMSE) or mean absolute error (MAE)? – Arguments against avoiding RMSE in the literature, Geosci. Model Dev., 7, 1247–1250, https://doi.org/10.5194/gmd-7-1247-2014, 2014.
Chang, L.-S., Schwartz, S. E., McGraw, R., and Lewis, E. R.: Sensitivity of aerosol properties to new particle formation mechanism and to primary emissions in a continental-scale chemical transport model, J. Geophys. Res.-Atmos., 114, D07203, https://doi.org/10.1029/2008JD011019, 2009.
Chen, G., Canonaco, F., Tobler, A., Aas, W., Alastuey, A., Allan, J., Atabakhsh, S., Aurela, M., Baltensperger, U., Bougiatioti, A., De Brito, J. F., Ceburnis, D., Chazeau, B., Chebaicheb, H., Daellenbach, K. R., Ehn, M., El Haddad, I., Eleftheriadis, K., Favez, O., Flentje, H., Font, A., Fossum, K., Freney, E., Gini, M., Green, D. C., Heikkinen, L., Herrmann, H., Kalogridis, A.-C., Keernik, H., Lhotka, R., Lin, C., Lunder, C., Maasikmets, M., Manousakas, M. I., Marchand, N., Marin, C., Marmureanu, L., Mihalopoulos, N., Močnik, G., Nęcki, J., O'Dowd, C., Ovadnevaite, J., Peter, T., Petit, J.-E., Pikridas, M., Matthew Platt, S., Pokorná, P., Poulain, L., Priestman, M., Riffault, V., Rinaldi, M., Różański, K., Schwarz, J., Sciare, J., Simon, L., Skiba, A., Slowik, J. G., Sosedova, Y., Stavroulas, I., Styszko, K., Teinemaa, E., Timonen, H., Tremper, A., Vasilescu, J., Via, M., Vodička, P., Wiedensohler, A., Zografou, O., Cruz Minguillón, M., and Prévôt, A. S. H.: European aerosol phenomenology - 8: Harmonised source apportionment of organic aerosol using 22 Year-long ACSM/AMS datasets, Environ. Int., 166, 107325, https://doi.org/10.1016/j.envint.2022.107325, 2022.
Chu, B., Kerminen, V.-M., Bianchi, F., Yan, C., Petäjä, T., and Kulmala, M.: Atmospheric new particle formation in China, Atmos. Chem. Phys., 19, 115–138, https://doi.org/10.5194/acp-19-115-2019, 2019.
Dada, L., Paasonen, P., Nieminen, T., Buenrostro Mazon, S., Kontkanen, J., Peräkylä, O., Lehtipalo, K., Hussein, T., Petäjä, T., Kerminen, V.-M., Bäck, J., and Kulmala, M.: Long-term analysis of clear-sky new particle formation events and nonevents in Hyytiälä, Atmos. Chem. Phys., 17, 6227–6241, https://doi.org/10.5194/acp-17-6227-2017, 2017.
Dada, L., Chellapermal, R., Buenrostro Mazon, S., Paasonen, P., Lampilahti, J., Manninen, H. E., Junninen, H., Petäjä, T., Kerminen, V.-M., and Kulmala, M.: Refined classification and characterization of atmospheric new-particle formation events using air ions, Atmos. Chem. Phys., 18, 17883–17893, https://doi.org/10.5194/acp-18-17883-2018, 2018.
Dada, L., Ylivinkka, I., Baalbaki, R., Li, C., Guo, Y., Yan, C., Yao, L., Sarnela, N., Jokinen, T., Daellenbach, K. R., Yin, R., Deng, C., Chu, B., Nieminen, T., Wang, Y., Lin, Z., Thakur, R. C., Kontkanen, J., Stolzenburg, D., Sipilä, M., Hussein, T., Paasonen, P., Bianchi, F., Salma, I., Weidinger, T., Pikridas, M., Sciare, J., Jiang, J., Liu, Y., Petäjä, T., Kerminen, V.-M., and Kulmala, M.: Sources and sinks driving sulfuric acid concentrations in contrasting environments: implications on proxy calculations, Atmos. Chem. Phys., 20, 11747–11766, https://doi.org/10.5194/acp-20-11747-2020, 2020.
Dal Maso, M., Kulmala, M., Riipinen, I., and Wagner, R.: Formation and growth of fresh atmospheric aerosols: Eight years of aerosol size distribution data from SMEAR II, Hyytiälä, Finland, Boreal Environ. Res., 10, 323–336, 2005.
Debevec, C., Sauvage, S., Gros, V., Sellegri, K., Sciare, J., Pikridas, M., Stavroulas, I., Leonardis, T., Gaudion, V., Depelchin, L., Fronval, I., Sarda-Esteve, R., Baisnée, D., Bonsang, B., Savvides, C., Vrekoussis, M., and Locoge, N.: Driving parameters of biogenic volatile organic compounds and consequences on new particle formation observed at an eastern Mediterranean background site, Atmos. Chem. Phys., 18, 14297–14325, https://doi.org/10.5194/acp-18-14297-2018, 2018.
Deng, C., Fu, Y., Dada, L., Yan, C., Cai, R., Yang, D., Zhou, Y., Yin, R., Lu, Y., Li, X., Qiao, X., Fan, X., Nie, W., Kontkanen, J., Kangasluoma, J., Chu, B., Ding, A., Kerminen, V.-M., Paasonen, P., Worsnop, D. R., Bianchi, F., Liu, Y., Zheng, J., Wang, L., Kulmala, M., and Jiang, J.: Seasonal Characteristics of New Particle Formation and Growth in Urban Beijing, Environ. Sci. Technol., 54, 8547–8557, https://doi.org/10.1021/acs.est.0c00808, 2020.
Deng, C., Cai, R., Yan, C., Zheng, J., and Jiang, J.: Formation and growth of sub-3 nm particles in megacities: impact of background aerosols, Faraday Discuss., 226, 348–363, https://doi.org/10.1039/D0FD00083C, 2021.
Ding, J., Dai, Q., Zhang, Y., Xu, J., Huangfu, Y., and Feng, Y.: Air humidity affects secondary aerosol formation in different pathways, Sci. Total Environ., 759, 143540, https://doi.org/10.1016/j.scitotenv.2020.143540, 2021.
Duplissy, J., Merikanto, J., Franchin, A., Tsagkogeorgas, G., Kangasluoma, J., Wimmer, D., Vuollekoski, H., Schobesberger, S., Lehtipalo, K., Flagan, R. C., Brus, D., Donahue, N. M., Vehkamäki, H., Almeida, J., Amorim, A., Barmet, P., Bianchi, F., Breitenlechner, M., Dunne, E. M., Guida, R., Henschel, H., Junninen, H., Kirkby, J., Kürten, A., Kupc, A., Määttänen, A., Makhmutov, V., Mathot, S., Nieminen, T., Onnela, A., Praplan, A. P., Riccobono, F., Rondo, L., Steiner, G., Tome, A., Walther, H., Baltensperger, U., Carslaw, K. S., Dommen, J., Hansel, A., Petäjä, T., Sipilä, M., Stratmann, F., Vrtala, A., Wagner, P. E., Worsnop, D. R., Curtius, J., and Kulmala, M.: Effect of ions on sulfuric acid-water binary particle formation: 2. Experimental data and comparison with QC-normalized classical nucleation theory, J. Geophys. Res.-Atmos., 121, 1752–1775, https://doi.org/10.1002/2015JD023539, 2016.
Eisele, F. L. and Tanner, D. J.: Measurement of the gas phase concentration of H2SO4 and methane sulfonic acid and estimates of H2SO4 production and loss in the atmosphere, J. Geophys. Res.-Atmos., 98, 9001–9010, https://doi.org/10.1029/93JD00031, 1993.
Glasoe, W. A., Volz, K., Panta, B., Freshour, N., Bachman, R., Hanson, D. R., McMurry, P. H., and Jen, C.: Sulfuric acid nucleation: An experimental study of the effect of seven bases, J. Geophys. Res. Atmospheres, 120, 1933–1950, https://doi.org/10.1002/2014JD022730, 2015.
Gordon, H., Kirkby, J., Baltensperger, U., Bianchi, F., Breitenlechner, M., Curtius, J., Dias, A., Dommen, J., Donahue, N. M., Dunne, E. M., Duplissy, J., Ehrhart, S., Flagan, R. C., Frege, C., Fuchs, C., Hansel, A., Hoyle, C. R., Kulmala, M., Kürten, A., Lehtipalo, K., Makhmutov, V., Molteni, U., Rissanen, M. P., Stozkhov, Y., Tröstl, J., Tsagkogeorgas, G., Wagner, R., Williamson, C., Wimmer, D., Winkler, P. M., Yan, C., and Carslaw, K. S.: Causes and importance of new particle formation in the present-day and preindustrial atmospheres, J. Geophys. Res.-Atmos., 122, 8739–8760, https://doi.org/10.1002/2017JD026844, 2017.
Hamed, A., Korhonen, H., Sihto, S.-L., Joutsensaari, J., Järvinen, H., Petäjä, T., Arnold, F., Nieminen, T., Kulmala, M., Smith, J. N., Lehtinen, K. E. J., and Laaksonen, A.: The role of relative humidity in continental new particle formation, J. Geophys. Res.-Atmos., 116, D03202, https://doi.org/10.1029/2010JD014186, 2011.
Hari, P. and Kulmala, M.: Station for measuring Ecosystem-Atmosphere relations (SMEAR II), Boreal Environ. Res., 10, 315–322, 2005.
He, X.-C., Tham, Y. J., Dada, L., Wang, M., Finkenzeller, H., Stolzenburg, D., Iyer, S., Simon, M., Kürten, A., Shen, J., Rörup, B., Rissanen, M., Schobesberger, S., Baalbaki, R., Wang, D. S., Koenig, T. K., Jokinen, T., Sarnela, N., Beck, L. J., Almeida, J., Amanatidis, S., Amorim, A., Ataei, F., Baccarini, A., Bertozzi, B., Bianchi, F., Brilke, S., Caudillo, L., Chen, D., Chiu, R., Chu, B., Dias, A., Ding, A., Dommen, J., Duplissy, J., El Haddad, I., Gonzalez Carracedo, L., Granzin, M., Hansel, A., Heinritzi, M., Hofbauer, V., Junninen, H., Kangasluoma, J., Kemppainen, D., Kim, C., Kong, W., Krechmer, J. E., Kvashin, A., Laitinen, T., Lamkaddam, H., Lee, C. P., Lehtipalo, K., Leiminger, M., Li, Z., Makhmutov, V., Manninen, H. E., Marie, G., Marten, R., Mathot, S., Mauldin, R. L., Mentler, B., Möhler, O., Müller, T., Nie, W., Onnela, A., Petäjä, T., Pfeifer, J., Philippov, M., Ranjithkumar, A., Saiz-Lopez, A., Salma, I., Scholz, W., Schuchmann, S., Schulze, B., Steiner, G., Stozhkov, Y., Tauber, C., Tomé, A., Thakur, R. C., Väisänen, O., Vazquez-Pufleau, M., Wagner, A. C., Wang, Y., Weber, S. K., Winkler, P. M., Wu, Y., Xiao, M., Yan, C., Ye, Q., Ylisirniö, A., Zauner-Wieczorek, M., Zha, Q., Zhou, P., Flagan, R. C., Curtius, J., Baltensperger, U., Kulmala, M., Kerminen, V.-M., Kurtén, T., Donahue, N. M., Volkamer, R., Kirkby, J., Worsnop, D. R., and Sipilä, M..: Role of iodine oxoacids in atmospheric aerosol nucleation, Science, 371, 589–595, https://doi.org/10.1126/science.abe0298, 2021.
Heinritzi, M., Dada, L., Simon, M., Stolzenburg, D., Wagner, A. C., Fischer, L., Ahonen, L. R., Amanatidis, S., Baalbaki, R., Baccarini, A., Bauer, P. S., Baumgartner, B., Bianchi, F., Brilke, S., Chen, D., Chiu, R., Dias, A., Dommen, J., Duplissy, J., Finkenzeller, H., Frege, C., Fuchs, C., Garmash, O., Gordon, H., Granzin, M., El Haddad, I., He, X., Helm, J., Hofbauer, V., Hoyle, C. R., Kangasluoma, J., Keber, T., Kim, C., Kürten, A., Lamkaddam, H., Laurila, T. M., Lampilahti, J., Lee, C. P., Lehtipalo, K., Leiminger, M., Mai, H., Makhmutov, V., Manninen, H. E., Marten, R., Mathot, S., Mauldin, R. L., Mentler, B., Molteni, U., Müller, T., Nie, W., Nieminen, T., Onnela, A., Partoll, E., Passananti, M., Petäjä, T., Pfeifer, J., Pospisilova, V., Quéléver, L. L. J., Rissanen, M. P., Rose, C., Schobesberger, S., Scholz, W., Scholze, K., Sipilä, M., Steiner, G., Stozhkov, Y., Tauber, C., Tham, Y. J., Vazquez-Pufleau, M., Virtanen, A., Vogel, A. L., Volkamer, R., Wagner, R., Wang, M., Weitz, L., Wimmer, D., Xiao, M., Yan, C., Ye, P., Zha, Q., Zhou, X., Amorim, A., Baltensperger, U., Hansel, A., Kulmala, M., Tomé, A., Winkler, P. M., Worsnop, D. R., Donahue, N. M., Kirkby, J., and Curtius, J.: Molecular understanding of the suppression of new-particle formation by isoprene, Atmos. Chem. Phys., 20, 11809–11821, https://doi.org/10.5194/acp-20-11809-2020, 2020.
Hellmuth, O.: Columnar modelling of nucleation burst evolution in the convective boundary layer – first results from a feasibility study Part I: Modelling approach, Atmos. Chem. Phys., 6, 4175–4214, https://doi.org/10.5194/acp-6-4175-2006, 2006.
Huijnen, V., Williams, J., van Weele, M., van Noije, T., Krol, M., Dentener, F., Segers, A., Houweling, S., Peters, W., de Laat, J., Boersma, F., Bergamaschi, P., van Velthoven, P., Le Sager, P., Eskes, H., Alkemade, F., Scheele, R., Nédélec, P., and Pätz, H.-W.: The global chemistry transport model TM5: description and evaluation of the tropospheric chemistry version 3.0, Geosci. Model Dev., 3, 445–473, https://doi.org/10.5194/gmd-3-445-2010, 2010.
Jiang, B. and Xia, D.: Role identification of NH3 in atmospheric secondary new particle formation in haze occurrence of China, Atmos. Environ., 163, 107–117, https://doi.org/10.1016/j.atmosenv.2017.05.035, 2017.
Jokinen, T., Sipilä, M., Junninen, H., Ehn, M., Lönn, G., Hakala, J., Petäjä, T., Mauldin III, R. L., Kulmala, M., and Worsnop, D. R.: Atmospheric sulphuric acid and neutral cluster measurements using CI-APi-TOF, Atmos. Chem. Phys., 12, 4117–4125, https://doi.org/10.5194/acp-12-4117-2012, 2012.
Jokinen, T., Lehtipalo, K., Thakur, R. C., Ylivinkka, I., Neitola, K., Sarnela, N., Laitinen, T., Kulmala, M., Petäjä, T., and Sipilä, M.: Measurement report: Long-term measurements of aerosol precursor concentrations in the Finnish subarctic boreal forest, Atmos. Chem. Phys., 22, 2237–2254, https://doi.org/10.5194/acp-22-2237-2022, 2022.
Junninen, H., Ehn, M., Petäjä, T., Luosujärvi, L., Kotiaho, T., Kostiainen, R., Rohner, U., Gonin, M., Fuhrer, K., Kulmala, M., and Worsnop, D. R.: A high-resolution mass spectrometer to measure atmospheric ion composition, Atmos. Meas. Tech., 3, 1039–1053, https://doi.org/10.5194/amt-3-1039-2010, 2010.
Kerminen, V.-M. and Kulmala, M.: Analytical formulae connecting the “real” and the “apparent” nucleation rate and the nuclei number concentration for atmospheric nucleation events, J. Aerosol Sci., 33, 609–622, https://doi.org/10.1016/S0021-8502(01)00194-X, 2002.
Kerminen, V.-M., Chen, X., Vakkari, V., Petäjä, T., Kulmala, M., and Bianchi, F.: Atmospheric new particle formation and growth: review of field observations, Environ. Res. Lett., 13, 103003, https://doi.org/10.1088/1748-9326/aadf3c, 2018.
Kiendler-Scharr, A., Wildt, J., Maso, M. D., Hohaus, T., Kleist, E., Mentel, T. F., Tillmann, R., Uerlings, R., Schurr, U., and Wahner, A.: New particle formation in forests inhibited by isoprene emissions, Nature, 461, 381–384, https://doi.org/10.1038/nature08292, 2009.
Kirkby, J., Curtius, J., Almeida, J., Dunne, E., Duplissy, J., Ehrhart, S., Franchin, A., Gagné, S., Ickes, L., Kürten, A., Kupc, A., Metzger, A., Riccobono, F., Rondo, L., Schobesberger, S., Tsagkogeorgas, G., Wimmer, D., Amorim, A., Bianchi, F., Breitenlechner, M., David, A., Dommen, J., Downard, A., Ehn, M., Flagan, R. C., Haider, S., Hansel, A., Hauser, D., Jud, W., Junninen, H., Kreissl, F., Kvashin, A., Laaksonen, A., Lehtipalo, K., Lima, J., Lovejoy, E. R., Makhmutov, V., Mathot, S., Mikkilä, J., Minginette, P., Mogo, S., Nieminen, T., Onnela, A., Pereira, P., Petäjä, T., Schnitzhofer, R., Seinfeld, J. H., Sipilä, M., Stozhkov, Y., Stratmann, F., Tomé, A., Vanhanen, J., Viisanen, Y., Vrtala, A., Wagner, P. E., Walther, H., Weingartner, E., Wex, H., Winkler, P. M., Carslaw, K. S., Worsnop, D. R., Baltensperger, U., and Kulmala, M.: Role of sulphuric acid, ammonia and galactic cosmic rays in atmospheric aerosol nucleation, Nature, 476, 429–433, https://doi.org/10.1038/nature10343, 2011.
Kulmala, M., Maso, M. D., Mäkelä, J. M., Pirjola, L., Väkevä, M., Aalto, P., Miikkulainen, P., Hämeri, K., and O'dowd, C. D.: On the formation, growth and composition of nucleation mode particles, Tellus B, 53, 479–490, https://doi.org/10.1034/j.1600-0889.2001.530411.x, 2001.
Kulmala, M., Vehkamäki, H., Petäjä, T., Dal Maso, M., Lauri, A., Kerminen, V.-M., Birmili, W., and McMurry, P. H.: Formation and growth rates of ultrafine atmospheric particles: a review of observations, J. Aerosol Sci., 35, 143–176, https://doi.org/10.1016/j.jaerosci.2003.10.003, 2004.
Kulmala, M., Lehtinen, K. E. J., and Laaksonen, A.: Cluster activation theory as an explanation of the linear dependence between formation rate of 3nm particles and sulphuric acid concentration, Atmos. Chem. Phys., 6, 787–793, https://doi.org/10.5194/acp-6-787-2006, 2006.
Kulmala, M., Petäjä, T., Nieminen, T., Sipilä, M., Manninen, H. E., Lehtipalo, K., Dal Maso, M., Aalto, P. P., Junninen, H., Paasonen, P., Riipinen, I., Lehtinen, K. E. J., Laaksonen, A., and Kerminen, V.-M.: Measurement of the nucleation of atmospheric aerosol particles, Nat. Protoc., 7, 1651–1667, https://doi.org/10.1038/nprot.2012.091, 2012.
Kulmala, M., Kontkanen, J., Junninen, H., Lehtipalo, K., Manninen, H. E., Nieminen, T., Petäjä, T., Sipilä, M., Schobesberger, S., Rantala, P., Franchin, A., Jokinen, T., Järvinen, E., Äijälä, M., Kangasluoma, J., Hakala, J., Aalto, P. P., Paasonen, P., Mikkilä, J., Vanhanen, J., Aalto, J., Hakola, H., Makkonen, U., Ruuskanen, T., Mauldin, R. L., Duplissy, J., Vehkamäki, H., Bäck, J., Kortelainen, A., Riipinen, I., Kurtén, T., Johnston, M. V., Smith, J. N., Ehn, M., Mentel, T. F., Lehtinen, K. E. J., Laaksonen, A., Kerminen, V.-M., and Worsnop, D. R.: Direct Observations of Atmospheric Aerosol Nucleation, Science, 339, 943–946, https://doi.org/10.1126/science.1227385, 2013.
Kulmala, M., Kerminen, V.-M., Petäjä, T., J. Ding, A., and Wang, L.: Atmospheric gas-to-particle conversion: why NPF events are observed in megacities?, Faraday Discuss., 200, 271–288, https://doi.org/10.1039/C6FD00257A, 2017.
Kulmala, M., Junninen, H., Dada, L., Salma, I., Weidinger, T., Thén, W., Vörösmarty, M., Komsaare, K., Stolzenburg, D., Cai, R., Yan, C., Li, X., Deng, C., Jiang, J., Petäjä, T., Nieminen, T., and Kerminen, V.-M.: Quiet New Particle Formation in the Atmosphere, Front. Environ. Sci., 10, 912385, https://doi.org/10.3389/fenvs.2022.912385, 2022a.
Kulmala, M., Cai, R., Stolzenburg, D., Zhou, Y., Dada, L., Guo, Y., Yan, C., Petäjä, T., Jiang, J., and Kerminen, V.-M.: The contribution of new particle formation and subsequent growth to haze formation, Environmental Science: Atmospheres, 2, 352–361, https://doi.org/10.1039/D1EA00096A, 2022b.
Kulmala, M., Cai, R., Ezhova, E., Deng, C., Stolzenburg, D., Dada, L., Guo, Y., Chao, Y., Peräkylä, O., Lintunen, A., Nieminen, T., Kokkonen, T. V., Sarnela, N., Petäjä, T., and Kerminen,V.-M.: Direct link between the characteristics of atmospheric new particle formation and Continental Biosphere-Atmosphere-Cloud-Climate (COBACC) feedback loop, Boreal Environ. Res., 28, 1–13, 2023.
Kürten, A., Rondo, L., Ehrhart, S., and Curtius, J.: Calibration of a Chemical Ionization Mass Spectrometer for the Measurement of Gaseous Sulfuric Acid, J. Phys. Chem. A, 116, 6375–6386, https://doi.org/10.1021/jp212123n, 2012.
Kyrö, E.-M., Väänänen, R., Kerminen, V.-M., Virkkula, A., Petäjä, T., Asmi, A., Dal Maso, M., Nieminen, T., Juhola, S., Shcherbinin, A., Riipinen, I., Lehtipalo, K., Keronen, P., Aalto, P. P., Hari, P., and Kulmala, M.: Trends in new particle formation in eastern Lapland, Finland: effect of decreasing sulfur emissions from Kola Peninsula, Atmos. Chem. Phys., 14, 4383–4396, https://doi.org/10.5194/acp-14-4383-2014, 2014.
Laakso, L., Petäjä, T., Lehtinen, K. E. J., Kulmala, M., Paatero, J., Hõrrak, U., Tammet, H., and Joutsensaari, J.: Ion production rate in a boreal forest based on ion, particle and radiation measurements, Atmos. Chem. Phys., 4, 1933–1943, https://doi.org/10.5194/acp-4-1933-2004, 2004.
Laarne, P., Amnell, E., Zaidan, M. A., Mikkonen, S., and Nieminen, T.: Exploring Non-Linear Dependencies in Atmospheric Data with Mutual Information, Atmosphere, 13, 1046, https://doi.org/10.3390/atmos13071046, 2022.
Lan, Z., Lin, W., Pu, W., and Ma, Z.: Measurement report: Exploring NH3 behavior in urban and suburban Beijing: comparison and implications, Atmos. Chem. Phys., 21, 4561–4573, https://doi.org/10.5194/acp-21-4561-2021, 2021.
Lee, S.-H., Gordon, H., Yu, H., Lehtipalo, K., Haley, R., Li, Y., and Zhang, R.: New Particle Formation in the Atmosphere: From Molecular Clusters to Global Climate, J. Geophys. Res.-Atmos., 124, 7098–7146, https://doi.org/10.1029/2018JD029356, 2019.
Lehtinen, K. E. J. and Kulmala, M.: A model for particle formation and growth in the atmosphere with molecular resolution in size, Atmos. Chem. Phys., 3, 251–257, https://doi.org/10.5194/acp-3-251-2003, 2003.
Lehtipalo, K., Yan, C., Dada, L., Bianchi, F., Xiao, M., Wagner, R., Stolzenburg, D., Ahonen, L. R., Amorim, A., Baccarini, A., Bauer, P. S., Baumgartner, B., Bergen, A., Bernhammer, A.-K., Breitenlechner, M., Brilke, S., Buchholz, A., Mazon, S. B., Chen, D., Chen, X., Dias, A., Dommen, J., Draper, D. C., Duplissy, J., Ehn, M., Finkenzeller, H., Fischer, L., Frege, C., Fuchs, C., Garmash, O., Gordon, H., Hakala, J., He, X., Heikkinen, L., Heinritzi, M., Helm, J. C., Hofbauer, V., Hoyle, C. R., Jokinen, T., Kangasluoma, J., Kerminen, V.-M., Kim, C., Kirkby, J., Kontkanen, J., Kürten, A., Lawler, M. J., Mai, H., Mathot, S., Mauldin, R. L., Molteni, U., Nichman, L., Nie, W., Nieminen, T., Ojdanic, A., Onnela, A., Passananti, M., Petäjä, T., Piel, F., Pospisilova, V., Quéléver, L. L. J., Rissanen, M. P., Rose, C., Sarnela, N., Schallhart, S., Schuchmann, S., Sengupta, K., Simon, M., Sipilä, M., Tauber, C., Tomé, A., Tröstl, J., Väisänen, O., Vogel, A. L., Volkamer, R., Wagner, A. C., Wang, M., Weitz, L., Wimmer, D., Ye, P., Ylisirniö, A., Zha, Q., Carslaw, K. S., Curtius, J., Donahue, N. M., Flagan, R. C., Hansel, A., Riipinen, I., Virtanen, A., Winkler, P. M., Baltensperger, U., Kulmala, M., and Worsnop, D. R.: Multicomponent new particle formation from sulfuric acid, ammonia, and biogenic vapors, Sci. Adv., 4, eaau5363, https://doi.org/10.1126/sciadv.aau5363, 2018.
Li, X.: Time series of J5 parameterization essential variables (MATLAB 2022b), Zenodo [data set/code], https://zenodo.org/records/15295592 (last access: 28 April 2025), 2025.
Li, X., Chee, S., Hao, J., Abbatt, J. P. D., Jiang, J., and Smith, J. N.: Relative humidity effect on the formation of highly oxidized molecules and new particles during monoterpene oxidation, Atmos. Chem. Phys., 19, 1555–1570, https://doi.org/10.5194/acp-19-1555-2019, 2019.
Liu, Q., Jia, X., Quan, J., Li, J., Li, X., Wu, Y., Chen, D., Wang, Z., and Liu, Y.: New positive feedback mechanism between boundary layer meteorology and secondary aerosol formation during severe haze events, Sci. Rep., 8, 6095, https://doi.org/10.1038/s41598-018-24366-3, 2018.
Liu, Y., Yan, C., Feng, Z., Zheng, F., Fan, X., Zhang, Y., Li, C., Zhou, Y., Lin, Z., Guo, Y., Zhang, Y., Ma, L., Zhou, W., Liu, Z., Dada, L., Dällenbach, K., Kontkanen, J., Cai, R., Chan, T., Chu, B., Du, W., Yao, L., Wang, Y., Cai, J., Kangasluoma, J., Kokkonen, T., Kujansuu, J., Rusanen, A., Deng, C., Fu, Y., Yin, R., Li, X., Lu, Y., Liu, Y., Lian, C., Yang, D., Wang, W., Ge, M., Wang, Y., Worsnop, D. R., Junninen, H., He, H., Kerminen, V.-M., Zheng, J., Wang, L., Jiang, J., Petäjä, T., Bianchi, F., and Kulmala, M.: Continuous and comprehensive atmospheric observations in Beijing: a station to understand the complex urban atmospheric environment, Big Earth Data, 4, 295–321, https://doi.org/10.1080/20964471.2020.1798707, 2020.
Määttänen, A., Merikanto, J., Henschel, H., Duplissy, J., Makkonen, R., Ortega, I. K., and Vehkamäki, H.: New Parameterizations for Neutral and Ion-Induced Sulfuric Acid-Water Particle Formation in Nucleation and Kinetic Regimes, J. Geophys. Res.-Atmos., 123, 1269–1296, https://doi.org/10.1002/2017JD027429, 2018.
Marten, R., Xiao, M., Rörup, B., Wang, M., Kong, W., He, X.-C., Stolzenburg, D., Pfeifer, J., Marie, G., Wang, D. S., Scholz, W., Baccarini, A., Lee, C. P., Amorim, A., Baalbaki, R., M. Bell, D., Bertozzi, B., Caudillo, L., Chu, B., Dada, L., Duplissy, J., Finkenzeller, H., Carracedo, L. G., Granzin, M., Hansel, A., Heinritzi, M., Hofbauer, V., Kemppainen, D., Kürten, A., Lampimäki, M., Lehtipalo, K., Makhmutov, V., Manninen, H. E., Mentler, B., Petäjä, T., Philippov, M., Shen, J., Simon, M., Stozhkov, Y., Tomé, A., Wagner, A. C., Wang, Y., Weber, S. K., Wu, Y., Zauner-Wieczorek, M., Curtius, J., Kulmala, M., Möhler, O., Volkamer, R., Winkler, P. M., Worsnop, D. R., Dommen, J., Flagan, R. C., Kirkby, J., Donahue, N. M., Lamkaddam, H., Baltensperger, U., and Haddad, I. E.: Survival of newly formed particles in haze conditions, Environmental Science: Atmospheres, 2, 491–499, https://doi.org/10.1039/D2EA00007E, 2022.
Martin, S. T., Artaxo, P., Machado, L. A. T., Manzi, A. O., Souza, R. A. F., Schumacher, C., Wang, J., Andreae, M. O., Barbosa, H. M. J., Fan, J., Fisch, G., Goldstein, A. H., Guenther, A., Jimenez, J. L., Pöschl, U., Silva Dias, M. A., Smith, J. N., and Wendisch, M.: Introduction: Observations and Modeling of the Green Ocean Amazon (GoAmazon2014/5), Atmos. Chem. Phys., 16, 4785–4797, https://doi.org/10.5194/acp-16-4785-2016, 2016.
Mauldin III, R. L., Frost, G. J., Chen, G., Tanner, D. J., Prevot, A. S. H., Davis, D. D., and Eisele, F. L.: OH measurements during the First Aerosol Characterization Experiment (ACE 1): Observations and model comparisons, J. Geophys. Res.-Atmos., 103, 16713–16729, https://doi.org/10.1029/98JD00882, 1998.
Mazon, S. B., Kontkanen, J., Manninen, H. E., Nieminen, T., Kerminen, V.-M., and Kulmala, M.: A long-term comparison of nighttime cluster events and daytime ion formation in a boreal forest, Boreal Environ. Res., 21, 242–261, 2016.
McMurry, P. H. and Friedlander, S. K.: New particle formation in the presence of an aerosol, Atmospheric Environment (1967), 13, 1635–1651, https://doi.org/10.1016/0004-6981(79)90322-6, 1979.
Myers, D. C., Kim, S., Sjostedt, S., Guenther, A. B., Seco, R., Vega Bustillos, O., Tota, J., Souza, R. A. F., and Smith, J. N.: Sulfuric acid in the Amazon basin: measurements and evaluation of existing sulfuric acid proxies, Atmos. Chem. Phys., 22, 10061–10076, https://doi.org/10.5194/acp-22-10061-2022, 2022.
Myllys, N., Kubečka, J., Besel, V., Alfaouri, D., Olenius, T., Smith, J. N., and Passananti, M.: Role of base strength, cluster structure and charge in sulfuric-acid-driven particle formation, Atmos. Chem. Phys., 19, 9753–9768, https://doi.org/10.5194/acp-19-9753-2019, 2019.
Neefjes, I., Laapas, M., Liu, Y., Medus, E., Miettunen, E., Ahonen, L., Quelever, L., Aalto, J., Bäck, J., Kerminen, V.-M., Lampilahti, J., Luoma, K., Mäki, M., Mammarella, I., Petäjä, T., Räty, M., Sarnela, N., Ylivinkka, I., Hakala, S., Kulmala, M., Nieminen, T., and Lintunen, A.: 25 years of atmospheric and ecosystem measurements in a boreal forest – Seasonal variation and responses to warm and dry years, Boreal Environ. Res., 27, 1–31, 2022.
Nieminen, T., Paasonen, P., Manninen, H. E., Sellegri, K., Kerminen, V.-M., and Kulmala, M.: Parameterization of ion-induced nucleation rates based on ambient observations, Atmos. Chem. Phys., 11, 3393–3402, https://doi.org/10.5194/acp-11-3393-2011, 2011.
Nieminen, T., Yli-Juuti, T., Manninen, H. E., Petäjä, T., Kerminen, V.-M., and Kulmala, M.: Technical note: New particle formation event forecasts during PEGASOS–Zeppelin Northern mission 2013 in Hyytiälä, Finland, Atmos. Chem. Phys., 15, 12385–12396, https://doi.org/10.5194/acp-15-12385-2015, 2015.
Nieminen, T., Kerminen, V.-M., Petäjä, T., Aalto, P. P., Arshinov, M., Asmi, E., Baltensperger, U., Beddows, D. C. S., Beukes, J. P., Collins, D., Ding, A., Harrison, R. M., Henzing, B., Hooda, R., Hu, M., Hõrrak, U., Kivekäs, N., Komsaare, K., Krejci, R., Kristensson, A., Laakso, L., Laaksonen, A., Leaitch, W. R., Lihavainen, H., Mihalopoulos, N., Németh, Z., Nie, W., O'Dowd, C., Salma, I., Sellegri, K., Svenningsson, B., Swietlicki, E., Tunved, P., Ulevicius, V., Vakkari, V., Vana, M., Wiedensohler, A., Wu, Z., Virtanen, A., and Kulmala, M.: Global analysis of continental boundary layer new particle formation based on long-term measurements, Atmos. Chem. Phys., 18, 14737–14756, https://doi.org/10.5194/acp-18-14737-2018, 2018.
NILU (Norwegian Institute for Air Research): EBAS database, http://ebas.nilu.no/, last access: 13 December 2024).
Okuljar, M., Kuuluvainen, H., Kontkanen, J., Garmash, O., Olin, M., Niemi, J. V., Timonen, H., Kangasluoma, J., Tham, Y. J., Baalbaki, R., Sipilä, M., Salo, L., Lintusaari, H., Portin, H., Teinilä, K., Aurela, M., Dal Maso, M., Rönkkö, T., Petäjä, T., and Paasonen, P.: Measurement report: The influence of traffic and new particle formation on the size distribution of 1–800 nm particles in Helsinki – a street canyon and an urban background station comparison, Atmos. Chem. Phys., 21, 9931–9953, https://doi.org/10.5194/acp-21-9931-2021, 2021.
Paasonen, P., Nieminen, T., Asmi, E., Manninen, H. E., Petäjä, T., Plass-Dülmer, C., Flentje, H., Birmili, W., Wiedensohler, A., Hõrrak, U., Metzger, A., Hamed, A., Laaksonen, A., Facchini, M. C., Kerminen, V.-M., and Kulmala, M.: On the roles of sulphuric acid and low-volatility organic vapours in the initial steps of atmospheric new particle formation, Atmos. Chem. Phys., 10, 11223–11242, https://doi.org/10.5194/acp-10-11223-2010, 2010.
Paulot, F., Crounse, J. D., Kjaergaard, H. G., Kürten, A., St. Clair, J. M., Seinfeld, J. H., and Wennberg, P. O.: Unexpected Epoxide Formation in the Gas-Phase Photooxidation of Isoprene, Science, 325, 730–733, https://doi.org/10.1126/science.1172910, 2009.
Petters, M. D. and Kreidenweis, S. M.: A single parameter representation of hygroscopic growth and cloud condensation nucleus activity, Atmos. Chem. Phys., 7, 1961–1971, https://doi.org/10.5194/acp-7-1961-2007, 2007.
Riccobono, F., Schobesberger, S., Scott, C. E., Dommen, J., Ortega, I. K., Rondo, L., Almeida, J., Amorim, A., Bianchi, F., Breitenlechner, M., David, A., Downard, A., Dunne, E. M., Duplissy, J., Ehrhart, S., Flagan, R. C., Franchin, A., Hansel, A., Junninen, H., Kajos, M., Keskinen, H., Kupc, A., Kürten, A., Kvashin, A. N., Laaksonen, A., Lehtipalo, K., Makhmutov, V., Mathot, S., Nieminen, T., Onnela, A., Petäjä, T., Praplan, A. P., Santos, F. D., Schallhart, S., Seinfeld, J. H., Sipilä, M., Spracklen, D. V., Stozhkov, Y., Stratmann, F., Tomé, A., Tsagkogeorgas, G., Vaattovaara, P., Viisanen, Y., Vrtala, A., Wagner, P. E., Weingartner, E., Wex, H., Wimmer, D., Carslaw, K. S., Curtius, J., Donahue, N. M., Kirkby, J., Kulmala, M., Worsnop, D. R., and Baltensperger, U.: Oxidation Products of Biogenic Emissions Contribute to Nucleation of Atmospheric Particles, Science, 344, 717–721, https://doi.org/10.1126/science.1243527, 2014.
Roldin, P., Swietlicki, E., Massling, A., Kristensson, A., Löndahl, J., Eriksson, A., Pagels, J., and Gustafsson, S.: Aerosol ageing in an urban plume – implication for climate, Atmos. Chem. Phys., 11, 5897–5915, https://doi.org/10.5194/acp-11-5897-2011, 2011.
Rönkkö, T., Kuuluvainen, H., Karjalainen, P., Keskinen, J., Hillamo, R., Niemi, J. V., Pirjola, L., Timonen, H. J., Saarikoski, S., Saukko, E., Järvinen, A., Silvennoinen, H., Rostedt, A., Olin, M., Yli-Ojanperä, J., Nousiainen, P., Kousa, A., and Dal Maso, M.: Traffic is a major source of atmospheric nanocluster aerosol, P. Natl. Acad. Sci. USA, 114, 7549–7554, https://doi.org/10.1073/pnas.1700830114, 2017.
Rosati, B., Christiansen, S., Wollesen de Jonge, R., Roldin, P., Jensen, M. M., Wang, K., Moosakutty, S. P., Thomsen, D., Salomonsen, C., Hyttinen, N., Elm, J., Feilberg, A., Glasius, M., and Bilde, M.: New Particle Formation and Growth from Dimethyl Sulfide Oxidation by Hydroxyl Radicals, ACS Earth Space Chem., 5, 801–811, https://doi.org/10.1021/acsearthspacechem.0c00333, 2021.
Ruosteenoja, K. and Räisänen, P.: Seasonal Changes in Solar Radiation and Relative Humidity in Europe in Response to Global Warming, J. Climate, 26, 2467–2481, https://doi.org/10.1175/JCLI-D-12-00007.1, 2013.
Salma, I. and Németh, Z.: Dynamic and timing properties of new aerosol particle formation and consecutive growth events, Atmos. Chem. Phys., 19, 5835–5852, https://doi.org/10.5194/acp-19-5835-2019, 2019.
Salma, I., Borsós, T., Weidinger, T., Aalto, P., Hussein, T., Dal Maso, M., and Kulmala, M.: Production, growth and properties of ultrafine atmospheric aerosol particles in an urban environment, Atmos. Chem. Phys., 11, 1339–1353, https://doi.org/10.5194/acp-11-1339-2011, 2011.
Salma, I., Németh, Z., Kerminen, V.-M., Aalto, P., Nieminen, T., Weidinger, T., Molnár, Á., Imre, K., and Kulmala, M.: Regional effect on urban atmospheric nucleation, Atmos. Chem. Phys., 16, 8715–8728, https://doi.org/10.5194/acp-16-8715-2016, 2016.
Salma, I., Thén, W., Aalto, P., Kerminen, V.-M., Kern, A., Barcza, Z., Petäjä, T., and Kulmala, M.: Influence of vegetation on occurrence and time distributions of regional new aerosol particle formation and growth, Atmos. Chem. Phys., 21, 2861–2880, https://doi.org/10.5194/acp-21-2861-2021, 2021.
Sanchez, K. J., Russell, L. M., Modini, R. L., Frossard, A. A., Ahlm, L., Corrigan, C. E., Roberts, G. C., Hawkins, L. N., Schroder, J. C., Bertram, A. K., Zhao, R., Lee, A. K. Y., Lin, J. J., Nenes, A., Wang, Z., Wonaschütz, A., Sorooshian, A., Noone, K. J., Jonsson, H., Toom, D., Macdonald, A. M., Leaitch, W. R., and Seinfeld, J. H.: Meteorological and aerosol effects on marine cloud microphysical properties, J. Geophys. Res.-Atmos., 121, 4142–4161, https://doi.org/10.1002/2015JD024595, 2016.
Schiro, K. A., Ahmed, F., Giangrande, S. E., and Neelin, J. D.: GoAmazon2014/5 campaign points to deep-inflow approach to deep convection across scales, P. Natl. Acad. Sci. USA, 115, 4577–4582, https://doi.org/10.1073/pnas.1719842115, 2018.
Sihto, S.-L., Kulmala, M., Kerminen, V.-M., Dal Maso, M., Petäjä, T., Riipinen, I., Korhonen, H., Arnold, F., Janson, R., Boy, M., Laaksonen, A., and Lehtinen, K. E. J.: Atmospheric sulphuric acid and aerosol formation: implications from atmospheric measurements for nucleation and early growth mechanisms, Atmos. Chem. Phys., 6, 4079–4091, https://doi.org/10.5194/acp-6-4079-2006, 2006.
Spracklen, D. V., Bonn, B., and Carslaw, K. S.: Boreal forests, aerosols and the impacts on clouds and climate, Philos. T. Roy. Soc. A, 366, 4613–4626, https://doi.org/10.1098/rsta.2008.0201, 2008.
Tunved, P., Hansson, H.-C., Kerminen, V.-M., Ström, J., Maso, M. D., Lihavainen, H., Viisanen, Y., Aalto, P. P., Komppula, M., and Kulmala, M.: High Natural Aerosol Loading over Boreal Forests, Science, 312, 261–263, https://doi.org/10.1126/science.1123052, 2006.
Tuovinen, S., Kontkanen, J., Jiang, J., and Kulmala, M.: Investigating the effectiveness of condensation sink based on heterogeneous nucleation theory, J. Aerosol Sci., 149, 105613, https://doi.org/10.1016/j.jaerosci.2020.105613, 2020.
Tuovinen, S., Cai, R., Kerminen, V.-M., Jiang, J., Yan, C., Kulmala, M., and Kontkanen, J.: Survival probabilities of atmospheric particles: comparison based on theory, cluster population simulations, and observations in Beijing, Atmos. Chem. Phys., 22, 15071–15091, https://doi.org/10.5194/acp-22-15071-2022, 2022.
Uno, I., Wang, Z., Itahashi, S., Yumimoto, K., Yamamura, Y., Yoshino, A., Takami, A., Hayasaki, M., and Kim, B.-G.: Paradigm shift in aerosol chemical composition over regions downwind of China, Sci. Rep., 10, 6450, https://doi.org/10.1038/s41598-020-63592-6, 2020.
Wang, M., Kong, W., Marten, R., He, X.-C., Chen, D., Pfeifer, J., Heitto, A., Kontkanen, J., Dada, L., Kürten, A., Yli-Juuti, T., Manninen, H. E., Amanatidis, S., Amorim, A., Baalbaki, R., Baccarini, A., Bell, D. M., Bertozzi, B., Bräkling, S., Brilke, S., Murillo, L. C., Chiu, R., Chu, B., De Menezes, L.-P., Duplissy, J., Finkenzeller, H., Carracedo, L. G., Granzin, M., Guida, R., Hansel, A., Hofbauer, V., Krechmer, J., Lehtipalo, K., Lamkaddam, H., Lampimäki, M., Lee, C. P., Makhmutov, V., Marie, G., Mathot, S., Mauldin, R. L., Mentler, B., Müller, T., Onnela, A., Partoll, E., Petäjä, T., Philippov, M., Pospisilova, V., Ranjithkumar, A., Rissanen, M., Rörup, B., Scholz, W., Shen, J., Simon, M., Sipilä, M., Steiner, G., Stolzenburg, D., Tham, Y. J., Tomé, A., Wagner, A. C., Wang, D. S., Wang, Y., Weber, S. K., Winkler, P. M., Wlasits, P. J., Wu, Y., Xiao, M., Ye, Q., Zauner-Wieczorek, M., Zhou, X., Volkamer, R., Riipinen, I., Dommen, J., Curtius, J., Baltensperger, U., Kulmala, M., Worsnop, D. R., Kirkby, J., Seinfeld, J. H., El-Haddad, I., Flagan, R. C., and Donahue, N. M.: Rapid growth of new atmospheric particles by nitric acid and ammonia condensation, Nature, 581, 184–189, https://doi.org/10.1038/s41586-020-2270-4, 2020.
Wang, M., Xiao, M., Bertozzi, B., Marie, G., Rörup, B., Schulze, B., Bardakov, R., He, X.-C., Shen, J., Scholz, W., Marten, R., Dada, L., Baalbaki, R., Lopez, B., Lamkaddam, H., Manninen, H. E., Amorim, A., Ataei, F., Bogert, P., Brasseur, Z., Caudillo, L., De Menezes, L.-P., Duplissy, J., Ekman, A. M. L., Finkenzeller, H., Carracedo, L. G., Granzin, M., Guida, R., Heinritzi, M., Hofbauer, V., Höhler, K., Korhonen, K., Krechmer, J. E., Kürten, A., Lehtipalo, K., Mahfouz, N. G. A., Makhmutov, V., Massabò, D., Mathot, S., Mauldin, R. L., Mentler, B., Müller, T., Onnela, A., Petäjä, T., Philippov, M., Piedehierro, A. A., Pozzer, A., Ranjithkumar, A., Schervish, M., Schobesberger, S., Simon, M., Stozhkov, Y., Tomé, A., Umo, N. S., Vogel, F., Wagner, R., Wang, D. S., Weber, S. K., Welti, A., Wu, Y., Zauner-Wieczorek, M., Sipilä, M., Winkler, P. M., Hansel, A., Baltensperger, U., Kulmala, M., Flagan, R. C., Curtius, J., Riipinen, I., Gordon, H., Lelieveld, J., El-Haddad, I., Volkamer, R., Worsnop, D. R., Christoudias, T., Kirkby, J., Möhler, O., and Donahue, N. M.: Synergistic HNO3–H2SO4–NH3 upper tropospheric particle formation, Nature, 605, 483–489, https://doi.org/10.1038/s41586-022-04605-4, 2022.
Wang, S., Peng, Y., Zhang, Q., Wang, W., and Wang, Q.: Mechanistic understanding of rapid H2SO4-HNO3-NH3 nucleation in the upper troposphere, Sci. Total Environ., 883, 163477, https://doi.org/10.1016/j.scitotenv.2023.163477, 2023.
Wang, Z. B., Hu, M., Yue, D. L., Zheng, J., Zhang, R. Y., Wiedensohler, A., Wu, Z. J., Nieminen, T., and Boy, M.: Evaluation on the role of sulfuric acid in the mechanisms of new particle formation for Beijing case, Atmos. Chem. Phys., 11, 12663–12671, https://doi.org/10.5194/acp-11-12663-2011, 2011.
Weber, R., Marti, J., McMurry, P., Eisele, F., Tanner, D., and Jefferson, A.: Measured atmospheric new particle formation rates: Implications for nucleation mechanisms, Chem. Eng. Commun., 151, 53–64, https://doi.org/10.1080/00986449608936541, 1996.
Xu, W., Gomez-Hernandez, M., Guo, S., Secrest, J., Marrero-Ortiz, W., Zhang, A. L., and Zhang, R.: Acid-Catalyzed Reactions of Epoxides for Atmospheric Nanoparticle Growth, J. Am. Chem. Soc., 136, 15477–15480, https://doi.org/10.1021/ja508989a, 2014.
Yan, C., Yin, R., Lu, Y., Dada, L., Yang, D., Fu, Y., Kontkanen, J., Deng, C., Garmash, O., Ruan, J., Baalbaki, R., Schervish, M., Cai, R., Bloss, M., Chan, T., Chen, T., Chen, Q., Chen, X., Chen, Y., Chu, B., Dällenbach, K., Foreback, B., He, X., Heikkinen, L., Jokinen, T., Junninen, H., Kangasluoma, J., Kokkonen, T., Kurppa, M., Lehtipalo, K., Li, H., Li, H., Li, X., Liu, Y., Ma, Q., Paasonen, P., Rantala, P., Pileci, R. E., Rusanen, A., Sarnela, N., Simonen, P., Wang, S., Wang, W., Wang, Y., Xue, M., Yang, G., Yao, L., Zhou, Y., Kujansuu, J., Petäjä, T., Nie, W., Ma, Y., Ge, M., He, H., Donahue, N. M., Worsnop, D. R., Kerminen, V.-M., Wang, L., Liu, Y., Zheng, J., Kulmala, M., Jiang, J., and Bianchi, F.: The Synergistic Role of Sulfuric Acid, Bases, and Oxidized Organics Governing New-Particle Formation in Beijing, Geophys. Res. Lett., 48, e2020GL091944, https://doi.org/10.1029/2020GL091944, 2021.
Yao, L., Garmash, O., Bianchi, F., Zheng, J., Yan, C., Kontkanen, J., Junninen, H., Mazon, S. B., Ehn, M., Paasonen, P., Sipilä, M., Wang, M., Wang, X., Xiao, S., Chen, H., Lu, Y., Zhang, B., Wang, D., Fu, Q., Geng, F., Li, L., Wang, H., Qiao, L., Yang, X., Chen, J., Kerminen, V.-M., Petäjä, T., Worsnop, D. R., Kulmala, M., and Wang, L.: Atmospheric new particle formation from sulfuric acid and amines in a Chinese megacity, Science, 361, 278–281, https://doi.org/10.1126/science.aao4839, 2018.
Yli-Juuti, T., Riipinen, I., Aalto, P. P., Nieminen, T., Maenhaut, W., Janssens, I. A., Claeys, M., Salma, I., Ocskay, R., Hoffer, A., Imre, K., and Kulmala, M.: Characteristics of new particle formation events and cluster ions at K-puszta, Hungary, Boreal Environ. Res., 14, 683–698, 2009.
Yu, F., Nadykto, A. B., Herb, J., Luo, G., Nazarenko, K. M., and Uvarova, L. A.: H2SO4–H2O–NH3 ternary ion-mediated nucleation (TIMN): kinetic-based model and comparison with CLOUD measurements, Atmos. Chem. Phys., 18, 17451–17474, https://doi.org/10.5194/acp-18-17451-2018, 2018.
Zaidan, M. A., Haapasilta, V., Relan, R., Paasonen, P., Kerminen, V.-M., Junninen, H., Kulmala, M., and Foster, A. S.: Exploring non-linear associations between atmospheric new-particle formation and ambient variables: a mutual information approach, Atmos. Chem. Phys., 18, 12699–12714, https://doi.org/10.5194/acp-18-12699-2018, 2018.
Zhang, Y., McMurry, P. H., Yu, F., and Jacobson, M. Z.: A comparative study of nucleation parameterizations: 1. Examination and evaluation of the formulations, J. Geophys. Res.-Atmos., 115, D20212, https://doi.org/10.1029/2010JD014150, 2010.
Zhao, B., Fast, J., Shrivastava, M., Donahue, N. M., Gao, Y., Shilling, J. E., Liu, Y., Zaveri, R. A., Gaudet, B., Wang, S., Wang, J., Li, Z., and Fan, J.: Formation Process of Particles and Cloud Condensation Nuclei Over the Amazon Rainforest: The Role of Local and Remote New-Particle Formation, Geophys. Res. Lett., 49, e2022GL100940, https://doi.org/10.1029/2022GL100940, 2022.
Zhou, Y., Hakala, S., Yan, C., Gao, Y., Yao, X., Chu, B., Chan, T., Kangasluoma, J., Gani, S., Kontkanen, J., Paasonen, P., Liu, Y., Petäjä, T., Kulmala, M., and Dada, L.: Measurement report: New particle formation characteristics at an urban and a mountain station in northern China, Atmos. Chem. Phys., 21, 17885–17906, https://doi.org/10.5194/acp-21-17885-2021, 2021.